Capstone Experience
Each academic year, industrial engineering and operations analytics seniors participate in the Industrial Engineering Capstone Experience as part of teams of four or five students. Every student pursuing the Bachelor of Science in Industrial Engineering and Operations Analytics at the University of Arkansas is required to complete the two-semester course sequence. Richard Cassady, university professor of industrial engineering, coordinates the Industrial Engineering Capstone Experience courses. The teams are matched with an industry partner and led by a student team leader.
In the fall semester, teams begin by getting to know their industry partner and the issues motivating the project. Students perform preliminary analysis and define objectives for their spring semester work. In the spring semester, the teams apply their industrial engineering and operations analytics skills to achieve their project objectives. They assess the potential impact of their work and create the deliverables that their industry partner need to implement their work.
The teams present their work at the Capstone Symposium in the spring, just before graduation. Industry partners and faculty mentors are recognized for their support of teams, and top teams and students receive awards in several categories.
The Department of Industrial Engineering at the University of Arkansas needs industry partners to support senior capstone projects. Each academic year, teams of four or five seniors will be assigned to one of the projects with one student serving as the team leader. The experience is coordinated by Richard Cassady (cassady@uark.edu), University Professor of Industrial Engineering, and students also receive technical advice from all industrial engineering faculty.
Formation of Teams
Dr. Cassady uses an application process to select the students who serve as team leaders. An auction-type process is then used to match each team leader with a project. Dr. Cassady assigns the remaining students to teams. Each team makes initial contact with their industry partner no later than the first week of September.
Intellectual Content of Projects
To provide a more specific description of the content of the capstone experience, nine specific capstone experience outcomes are used to guide the activities that are part of the experience.
Although every project is different, and all projects have evolving requirements:
- Each team will describe the mission of their industry partner organization. For large organizations, the team will describe the mission of the segment(s) of the organization that is of specific interest to the project.
- Each team will describe: (a) the concerns of their industry partner organization that are motivating their project; and (b) any formal and/or ad hoc processes, procedures, policies, etc., that are currently in place and are related to the concerns motivating their project.
- Each team will: (a) identify and/or define one or more metrics that can be used to determine their success in addressing the concerns motivating their project; and (b) attempt to assess and evaluate how their industry partner organization currently is performing relative to the project-success metrics.
- Each team will make recommendations for addressing the concerns motivating their project and use the project-success metrics to assess and evaluate these recommendations.
- Each team will provide deliverables to their industry partner organization that facilitate the implementation of their recommendations.
In achieving capstone experience outcomes 1-5:
- Each team will use project management software to track and document their activities and hold frequent meetings to receive support and feedback from their industry partner organization and the faculty coordinator.
- Each team will: (a) make use of the academic literature related to their project; and (b) learn and apply industrial engineering and operations analytics skills beyond their required BSIEOA coursework.
During each semester:
- Each team will communicate their findings in: (a) well-organized and well-written documents; and (b) well-organized, well-rehearsed and effective oral communication.
Throughout the experience:
- Each student will demonstrate professionalism and effective teamwork.
Expectations of Industry Partners
A successful capstone project requires extensive and timely support from the industry partner. The industry partner is expected to provide the team with a point of contact (and a backup point of contact) who can meet with the team (in-person or remotely) at least every other week to help the team identify and interview stakeholders, obtain data, assess the validity of their analysis, review course milestones and project deliverables, etc. The industry partner is also expected to cover any travel expenses incurred by the team during the project and to participate in the team’s final presentation near the end of the project.
Industry partners are expected to provide project ideas that are consistent with the department’s expectations regarding technical content. Historically, meeting teams’ data needs has been the most difficult expectation for industry partners to meet. Industry partners should consider the availability of real and relevant data when submitting project ideas. While the department is not opposed to teams collecting data as part of their project activities, the preference is that the majority of project activities related to data be focused on analysis rather than collection. Specifically, the preference is that projects require teams to deal with large, messy, data sets.
Limited scope also has been a challenge associated with some past projects. For example, developing a forecasting tool or developing a software tool to automate a manual process are worthy project activities. However, neither is enough on its own to meet the department’s expectations for a capstone project. The department wants students to take the next step to understand how the predictive model or the software tool impacts strategic or tactical decision-making.
Dissemination
Once a potential industry partner expresses interest, issues related to non-disclosure and intellectual property will be addressed via a separate email communication. When necessary, the university and the industry partner enter into an agreement that covers non-disclosure and intellectual property. The agreement covers all university faculty and staff. Student teams sign a read-and-acknowledge form related to this agreement.
The audience for almost all capstone experience documents and presentations is limited to the industrial engineering faculty and staff, the students in the capstone experience and the industry partner associated with the project being discussed. However, there are a few public activities associated with the capstone experience. First, teams make a final, fall semester presentation that is open to the entire industrial engineering student body. The industry partner has the responsibility of approving any information that their student team disseminates in this presentation.
Second, the experience concludes with the Industrial Engineering Capstone Symposium in late April or early May. At the symposium, which will be open to the public, teams participate in an interactive poster session and deliver detailed technical presentations about their projects. The industry partner has the responsibility of approving any information that their student team disseminates at the symposium.
Third, at least one team will be selected to compete for the Institute of Industrial and Systems Engineers (IISE) Senior Capstone Design Award. To enter this competition, each selected team submits a written executive summary, a poster and a set of presentation slides regarding their project.
Typical Milestones
The following list summarizes the major milestones associated with the experience. Additional details are provided to industry partners throughout the experience.
Early September | Teams contact industry partner for the first time |
Early November | Industry partner submits team assessment 1 |
Late November | Industry partner submits team assessment 2 |
Late November | Industry partner approves fall, public presentation content |
Early December | Teams make fall, public presentations |
Mid-February | Industry partner submits team assessment 3 |
Mid-March | Industry partner submits team assessment 4 |
Mid-March | Industry partner approves team’s symposium website material |
Mid-March | Industry partner agrees to list of final project deliverables |
Late April | Industry partner submits ABET assessment |
Late April | Industry partner approves public symposium content |
Late April | Industry partner approves final project deliverables |
Late April | Annual IE Capstone Symposium |
Submission of Project Ideas
If you are interested in being one of our industry partners, please contact Richard Cassady at cassady@uark.edu. Dr. Cassady is willing to meet with you (in-person or remotely) to discuss the capstone experience in more detail. Each year, our goal is to have our industry partners identified by mid-June.
8th Annual Industrial Engineering Capstone Symposium
Arkansas Union Verizon Ballroom, University of Arkansas, Fayetteville
Wednesday, May 1, 2024
Forecasting Backlog of Cross-Dock Operations using Regression and Machine Learning Algorithms
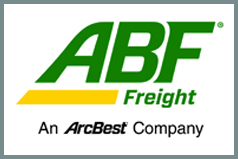
Student Team Leader: Marshal Ray
Other Student Team Members: Maryanne Attee, Jacob Dixon, James Jett and Seth Turner
Industry Partner: ABF Freight
Industry Partner Contacts: Bradley Taylor, Lead Operations Strategy Engineer; Chad Treadaway, Senior Manager, Engineering
ABF Freight, a subsidiary of ArcBest, excels in nationwide less-than-truckload (LTL) shipping, with operations based in Fort Smith, AR. Its vast network includes 10 distribution centers and 240 service centers, handling approximately 20,500 daily shipments in 2023 (8K released in February 2024). ABF Freight is known for providing efficient and cost-effective shipping solutions. However, ABF Freight faces complexities in managing its cross-dock operations due to factors such as equipment availability, labor, freight profiles, and fluctuating demand, which pose logistical challenges at these hubs. We primarily focused on addressing fluctuations in demand. ABF Freight utilizes a metric called Days-To-Current (DTC) to assess how swiftly a station can clear its inventory. Following an analysis of extensive freight data, we explored various regression and machine learning techniques to forecast DTC five days into the future. Subsequently, we developed a Python-based decision support tool incorporating our most successful models. This tool generates a forecasted DTC value for each distribution center for the next five days, empowering ABF Freight to make preemptive adjustments to their network, potentially reducing backlogs, minimizing costs, and optimizing profitability.
Designing a User interface with Excel VBA to Generate Rate Increases for Existing Low-Risk Accounts
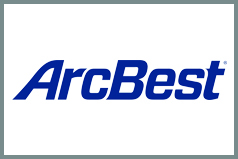
Student Team Leader: Ellen Timmerman
Other Student Team Members: Natalie Hernandez, Kaden Jones and Elias Theodore
Industry Partner: ArcBest
Industry Partner Contacts: Drew Caple, Manager of Pricing and Supply Chain Analytics; Erin Hills, Logistics Engineer II
ArcBest, headquartered in Fort Smith, AR, is a five-billion-dollar integrated logistics corporation with multiple subsidiaries. ABF Freight is by far the largest of their subsidiaries, accounting for over 60% of ArcBest’s annual revenue, and specializes in less-than-truckload shipments. While ABF has standard base rates available to any customer, preferred customers may negotiate personalized pricing structures. Each customer's pricing undergoes an annual review to ensure profitability, with adjustments made based on market changes and cost fluctuations. However, the process of evaluating and adjusting pricing for smaller customers can be disproportionately time-consuming for pricing analysts compared to the revenue generated from those accounts. To address this, we aim to streamline rate increase tasks for smaller accounts by developing a decision-support tool with an intuitive user interface that automatically generates rate increases, considering past account performance. Using the ROMC framework for specialized decision support systems, we created our tool in Excel's VBA. This interface enables analysts to quickly make informed decisions about each rate increase task by assessing an account's historical profitability and predicted negotiation style.
Improving Radiology Productivity by Optimizing Planned Staffing Levels and Supporting Real-Time Adjustments
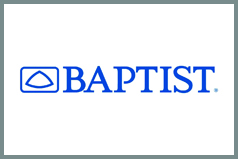
Student Team Leader: Karleigh Eoff
Other Student Team Members: Charles Eason, Weston Harp, Du'Maurier Looney and Joshua Peetoom
Industry Partner: Baptist Memorial Healthcare Corporation
Industry Partner Contacts: Katie Parker, System Director for Human Resources
Headquartered in Memphis, Tennessee, Baptist Memorial Healthcare Corporation (BMHCC) is a network of hospitals and healthcare facilities that serve the Mid-South Region. We are working with BMHCC’s Women’s Hospital’s radiology department in Memphis, TN. The radiology department struggled to meet productivity goals set by BMHCC in the 2023 fiscal year. We are focusing on the staffing of radiology technicians to improve the department's productivity. Currently, there are baseline staff levels for each type of technician (MRI, CT, Ultrasound, and X-Ray) for each day of the week, but these baseline numbers can be adjusted throughout the day by managers if needed. First, we created an optimization model to adjust the baseline staff levels for each type of technician in the department. The model minimizes labor hours of the technicians while meeting demand and labor requirements set by BMHCC. We created a decision support tool to aid in decisions regarding staff levels throughout the day. This tool allows the managers to get an estimate of what productivity is currently and how adjusting the current staff level will affect productivity.
Applying Markov Decision Processes to Optimize Aggregate Workforce Planning with Uncertain Funding Conditions
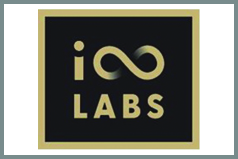
Student Team Leader: Blake Sooter
Other Student Team Members: Katie Augsburger, James Fite, Nathanael Harris and Deniz Vural
Industry Partner: Infinity Labs LLC.
Industry Partner Contacts: Stephen Sieck, Division Lead, Transformational Technologies; David Hillstrom, Ph.D., Simulation Scientist
Founded in 2020 with 7 employees, Infinity Labs is an innovation-focused firm that fosters an entrepreneurial mindset to solve the world’s hardest problems. Located in Dayton, Ohio, Infinity Labs specializes in modeling and simulation work, primarily in the defense sector. Accelerating growth at the company has led to a litany of challenges relating to hiring and staffing, and Infinity desires a more robust quantitative component to their decision-making process for hiring new technical staff. Our team has improved Infinity’s full-time equivalent (FTE) need forecast by better accounting for uncertain inputs using Monte Carlo simulation. We then use this estimate to optimize sequential hiring decisions, which we’ve modeled as a Markov Decision Process. We formulated our model such that the observable state is the gap between actual and needed FTEs at a given step in time, which then prescribes an aggregate hiring action to the decision maker. Our team is developing a decision support tool in Python that will include Excel input/output of project data, the ability to modify assumptions such as the possible hiring decisions, distribution assumptions, discount factor, etc., and the ability to measure changes’ impact on model results.
Delivery Zone Definition with K-Medoids Clustering and Integer Programming
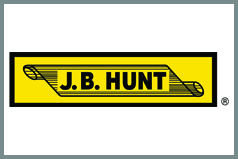
Student Team Leader: Rachel Thomas
Other Student Team Members: Zayna Abu-Safe, Bennett Foret, Trent Sawyer and Luke Smith
Industry Partner: J.B. Hunt
Industry Partner Contacts: Reid Nelson, Manager II, E&T; Brett Phillippe, Sr. Logistics Engineer
J.B. Hunt is a Fortune 500 transportation logistics company that provides shipping and carrier solutions to its customers. With over 35,000 employees, J.B. Hunt has five business units, including Final Mile, who specializes in delivering products to customers via freight. J.B. Hunt Final Mile defines delivery zones for several of its customers to reduce their weekly mileage. A delivery zone is a group of zip codes serviced on specific day(s) of the week. J.B. Hunt Final Mile Engineering’s challenge is that its current process of creating delivery zones is a manual, guess-and-check process that is time-consuming and does not guarantee the best zone definition for reducing route mileage. To address this, we developed a decision-support tool that uses Python to automate and improve the process of creating delivery zones. Using K-Medoids clustering and an integer program, we devised a comprehensive solution to streamline delivery zone creation. We determine the number of zones to create for a customer account, assign zip codes to zones, and assign delivery days to zones. The primary goal is to create zone definitions where workload and daily volume are balanced throughout the week and average weekly miles per stop for customer accounts are reduced.
Allocating Truck Yard Resources and Reducing Bobtail Miles
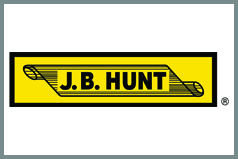
Student Team Leader: Kate Bowden
Other Student Team Members: Hector D. Santillan, Mackenzie Frederick, Riley Baker and Santiago Schrader
Industry Partner: J.B. Hunt Transport Services, Inc.
Industry Partner Contacts: Jake Wofford, Vice President, Intermodal Operations; Trevor Rogers, Director II, Operations and Brandon Workman, Logistics Engineer II, West Dray Operations
J.B. Hunt Transport Services, Inc., a Fortune 500 company headquartered in Lowell, Arkansas, provides trucking and logistics services across five business units. The Intermodal business unit utilizes a network of five truck yards and the BNSF Railway to deliver freight in the Los Angeles, California, region. J.B. Hunt is concerned that they are driving too many "bobtail" miles, which occurs when there is no trailing equipment attached to the cab of a truck. Bobtailing typically occurs at the beginning and end of a driver's shift, driving between a truck yard and a rail yard. J.B. Hunt recently purchased two new truck yards in Los Angeles and believes the properties' proximity to the rail yards can decrease bobtailing. We developed an optimization model that minimized bobtailing by assigning trucks to yards, giving us a baseline recommendation for the resource allocation at the new truck yards. For the remaining space at the truck yards, we created a VBA decision support tool allowing users to rank the relative importance of dedicating space for trucks, trailers, containers, and office space at each new yard. The tool performs a multiple objective decision analysis, outputting the recommended amount of equipment to store at each yard.
Improving Table-Pack Work Cell Efficiency using Intrinsic Motivation and Lean Manufacturing Principles
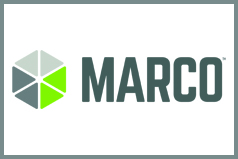
Student Team Leader: Gabe Hesington
Other Student Team Members: Joshua Jowers, Caleb Morris and Mitchell Cooksey
Industry Partner: Marco Group, Inc.
Industry Partner Contacts: Richard Davidson, Chief Executive Officer, Co-Founder
Marco Group, Inc. is a manufacturer of classroom furniture that is headquartered in Neosho, MO. They employ about 65 people and generate around $20 million in annual revenue. Marco uses cellular manufacturing to produce a wide array of desks and tables, while outsourcing production of chairs and storage carts. The last work cell that all tables go through is called Table-Pack, where each tabletop is inspected, cleaned, given support braces if needed, and packaged. We discovered that Table-Pack’s performance was substantially lower than Marco’s standardized goals. We attributed this to several factors including lack of standard practices, Parkinson’s law, and a lack of employee motivation. We have outlined a series of standard practices using Standard Operating Procedures (SOP) documentation. These documents outline safe work standards covering cell layout, work instructions, and lean manufacturing techniques. These documents lay a foundation for improved process observation and future improvements. To address Parkinson’s law and employee motivation, we built an Operator Dashboard that gives workload visibility and specific goals to the Table-Pack operators. The goal of the dashboard is to give operators intrinsic motivation which, based on our research, increases productivity.
Improving Chicken Breast Production Scheduling through Pareto Optimization with VB.NET
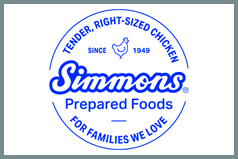
Student Team Leader: Addison Standridge
Other Student Team Members: Carter Baldwin, Kevin Connelly and Joshua Smith
Industry Partner: Simmons Prepared Foods
Industry Partner Contacts: Turner Vance, Director Continuous Improvement; Ross Vandever, VP Continuous Improvement; Kerry Bartholomew, Senior Continuous Improvement Manager
Headquartered in Siloam Springs, Arkansas, Simmons Foods, Inc. and its associated enterprises are primary providers of poultry, pet, and animal nutrition items. Simmons Prepared Foods ranks among the top 15 poultry producers in the United States and operates as a division of Simmons Foods within the foodservice industry. A variety of people, starting with the sales team all the way to the plant manager, give different inputs to generate a customer order schedule. This schedule begins with forecasts from the sales team and then each plant scheduler generates a schedule with these inputs for their respective facility based on long-term employee knowledge. Currently, there is no process during schedule building that incorporates the minimization of waste of value-added product, or trim. Our primary goal is to use multi-objective optimization to improve the production schedule for chicken breasts at Simmons’ Van Buren facility with an aim to enhance the output of value-added product while simultaneously reducing the tardiness of customer orders. We designed a Microsoft Excel Add-In tool using Microsoft Visual Basic .NET framework that allows Simmons to choose from multiple solutions on a Pareto frontier. This increases the flexibility of the scheduler’s decision, speeds up the time to generate a schedule, and incorporates trim reduction into the schedule building process.
Enhancing Automation Decisions using Power BI Analysis and Linear Programming
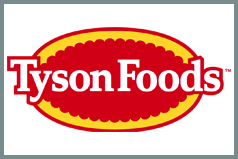
Student Team Leader: Lathan Gregg
Other Student Team Members: Ashwin Narayan, Austin Easley, Seth Rosenfeld and Sebastian Alborta
Industry Partner: Tyson Foods
Industry Partner Contacts: Maria Rene Arandia, Automation Analyst; Irving Polanco, Senior Automation & Robotics Engineer; Sean Lawrence, Senior Manager Automation
Tyson Foods, headquartered in Springdale, Arkansas, is a Fortune 100 multinational protein corporation serving consumers on five continents. Tyson divides their operations into three business units: Poultry, Prepared Foods, and Fresh Meats. Tyson’s automation team works across all three units to deliver automated solutions, helping reduce costs and eliminate ergonomic risks. Automation engineers analyze end of line data collected inside facilities to understand the volume and variety of products handled. At Fresh Meats facilities, non-uniform and decentralized data complicates this process, resulting in a tedious and incomprehensive ad hoc analysis. Our project goal was to streamline this process while providing a more complete analysis of facility data. To accomplish this, we created an ingestion process to load then structure data, and an interactive Power BI dashboard to support the comprehensive examination of a facility’s performance. To supplement this tool, we created a linear program to prescribe an optimal combination of palletizer options to implement at a facility.
7th Annual Industrial Engineering Capstone Symposium
Arkansas Union Verizon Ballroom, University of Arkansas, Fayetteville
Wednesday, May 3, 2023
Improving Starting Price Point for Customer Re-Negotiations using XGBoost
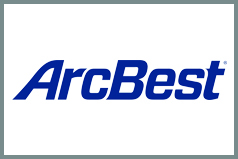
Student Team Leader: Abby Harris
Other Student Team Members: Zachary Oldham, Wesley Tate, Ernesto Serna and Jacob Boshears
Industry Partner: ArcBest
Industry Partner Contacts: Drew Caple, Manager - Pricing and Supply Chain Analytics; Erin Hills, Logistics Engineer II
ArcBest is a five-billion-dollar transportation and logistics company based in Fort Smith, AR. ABF Freight is ArcBest’s largest subsidiary and specializes in less-than-truckload (LTL) shipments, employing thousands of people. Roughly 50% of ABF’s clients use pricing structures that expire after one year, triggering a two-and-a-half-month re-negotiation process. This process is currently based heavily on an engineer’s experience or knowledge about a customer. The goal of our project is to find a way to streamline and standardize the beginning of this process by setting the initial price increase. Using past re-negotiation data provided by ArcBest, we grouped instances of customer re-negotiations into bins allowing XGBoost models to be built that predict the amount customers will negotiate. We then packaged these predictive models into an easy-to-use tool in R that allows an engineer to input customer account information and receive a starting point for their negotiations. This tool is expected to reduce the time it takes an engineer to complete the overall re-negotiation process.
Reducing Stock-outs in a Hospital Medication Dispensing System using Simulation Analysis
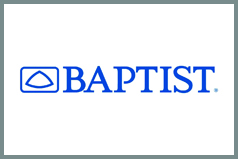
Student Team Leader: Carson Doss
Other Student Team Members: Armon Afrasiabi, Gabe Ellis, Will Plunkett and Dylan Deramus
Industry Partner: Baptist Memorial Health Care Corporation
Industry Partner Contacts: Katie Parker, Director of Performance Improvement; and Ben Eddlemon, Director of Pharmacy at DeSoto Hospital
Baptist Memorial Health Care Corporation (BMHCC) is network of hospitals spanning the greater Memphis area that started in 1912. Their network includes 22 hospitals with over 600 of the region’s leading specialists. Our project focuses on the Omnicell Medication Dispensing Cabinets within the DeSoto Hospital located in Southaven, Mississippi. BMHCC uses Omnicell cabinets to hold and dispense drugs to nurses and staff as needed. These Omnicell cabinets use different par levels for each medication stored within them. When a medication is at ‘par’, this means that the stock for the respective medication is full. However, a medication could completely stock-out, meaning its stock is at zero. BMHCC made it our focus to analyze different stock levels to work to minimize these stockouts. We created an Omnicell simulation in Arena. With our process analyzer, we were able to evaluate many combinations of par level versus demand levels. The results of the simulation populate a visual to prescribe par levels based on demand level and desired fill rate.
A Decision Support Tool to Automate and Optimize Contract Staffing using Linear Programming
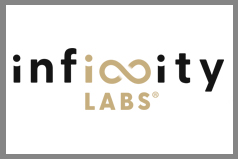
Student Team Leader: Paris Joslin
Other Student Team Members: Nathan Skinner, Will Cunningham, Conner Oxford and Zach Leondike
Industry Partner: Infinity Labs LLC
Industry Partner Contacts: Stephen Sieck, Senior Program Manager; and Nick Marquart, Co-Founder and Chief Analytics Officer
Infinity Labs LLC is a cutting-edge innovation company headquartered in Dayton, Ohio, that specializes in utilizing innovative modeling and simulation, cybersecurity, and training techniques to solve the hardest problems in the national defense industry. Since the launch of the company in 2020, Infinity Labs has experienced rapid growth with employees located across the United States. Due to the increasing number of employees and the complex nature of defense contracts, the staffing process has become time-consuming and requires a significant amount of effort from program managers. Infinity Labs has expressed the need for an automated tool that alleviates time constraints on program managers and ultimately balances employee utilizations. Our team designed a Microsoft Excel decision support tool that analyzes employee qualifications and contract requirements. The goal of our optimization model is to minimize the variability in employee utilizations in order to create a balanced workforce. The tool utilizes AMPL to solve a linear programming model to produce optimal staffing assignments. Using our tool, program managers are able to post-process the optimal assignments to fit business needs for the future months.
Developing a Priority Assignment Policy for the Empty Planner Application
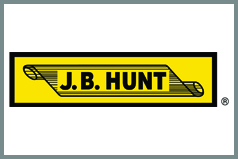
Student Team Leader: Bill Byers
Other Student Team Members: Jackson Barclay, Carter Christian and Joshua Walters
Industry Partner: J.B. Hunt Transport Services, Inc.
Industry Partner Contacts: Jessica Smith, Sr. Manager, Engineering & Technology; Sam Webb, Data Scientist, Engineering & Technology
J.B. Hunt Transport Services, Inc., is a Fortune 500 company located in Lowell, Arkansas, that provides a variety of freight transportation services for customers. J.B. Hunt has five different business units in operation: Intermodel (JBI), Dedicated Contacted Services (DCS), Integrated Capacity Solutions (ICS), Final Mile, and Truckload (JBT). For this project, we are focusing on supporting the decisions derived from an application developed by J.B. Hunt to aid dispatchers in the assignment of empty equipment throughout their daily operations. We did this by assigning numeric scores to each potential location to provide insight for where to assign drivers. We used Multi-Objective Decision Analysis (MODA) to understand the importance of different factors that are a part of the policy that we used to score each location and created a swing weight matrix to weigh the factors using stakeholder analysis. We reinforced the improvement of our metric of Unplanned Empty Moves with the use of simulation that created a baseline to show the current performance without the use of our policy. We then compared those results to another simulation that incorporates our policy to capture potential improvements. Finally, we created a VBA-based decision support tool that allows users to interact with the policy in real time to understand its outputs.
Linear Optimization for Driver-Route Assignment with Fatigue and Balanced Workload Considerations
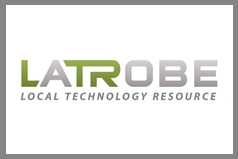
Student Team Leader: Andrew Freeman
Other Student Team Members: Katy Emerson, Nicolas Alcoreza, Sarah Wilson and Emmanuel Jean Paul De La Gala Gomez
Industry Partner: LATROBE LLC
Industry Partner Contacts: Latanyua Robinson, President and James Robinson, Vice-President
Latrobe LLC, founded in 2010 and headquartered in Memphis, Tennessee specializes in manufacturing consulting, light assembly and packaging, and shipping operations as an Amazon Delivery Service Partner (DSP). Latrobe’s DSP division is responsible for hiring, training, and assigning drivers their daily routes. Currently, Amazon provides a random initial daily assignment of drivers to routes. Latrobe’s coordinator makes changes based on their knowledge to improve the assignment each morning. Routes have a wide range of difficulty and poor assignments can cause the dual problem of underworked drivers and fatigued drivers. Our goal was to build a model that automatically highlights changes to reduce fatigue by balancing the workload fairly among drivers. We defined a linear program that considers the number of packages a driver delivered in the past few days as a measure of the driver’s fatigue. The model minimizes the deviation between each driver’s workload and the system average. The linear program was defined in Python and displayed in a user interface in PowerBI. The optimization model and visuals in the dashboard also provide the company with great insight into past performance and future improvement recommendations.
Analyzing and Predicting Marketing Campaign Performance Using Regression and Time Series Analysis
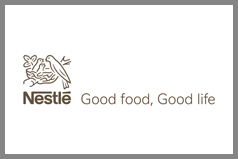
Student Team Leader: Agustin German Reichhardt
Other Student Team Members: Luke Tyler, Sam Nelson, Sebastian Alborta and Skyler Mantooth
Industry Partner: Nestlé
Industry Partner Contacts: Edosa Aibangbee - Manager, Customer eCommerce Account, Walmart Frozen; Megan McCoy - Category Manager, Strategic Planning and Insights, Walmart and Kara Puntriano - Senior Analyst Category Management
We partnered with Nestle’s Rogers, Arkansas, office to perform descriptive and predictive analysis on the sales of Nestlé USA brands and products such as DiGiorno frozen pizza. The project aimed to analyze sales and marketing data from 2019 to 2023. The sales data contained the dollar sales from 2019 to 2023 of each product sold by more than ten different brands, and the marketing data contained information on when and where advertisements for each specific brand were launched. The data also contained the media type of each advertisement. Using this information, we identified four different areas that could impact the ad performance in terms of sales, which include the media type of the ad, the time of launch of the ad, if competition ads were active at the same time, and if there was a major event active during the time the ad was running. With modern statistical techniques such as regression and time series analysis, we created a final report that will provide insight on the effectiveness and impact of each ad campaign, including media types and month of launch, that Nestlé can use when planning future ad campaigns.
Increasing Production Capacity and Efficiency through Technology Upgrades and Labor Allocation
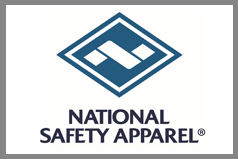
Student Team Leader: Ashley Stanek
Other Student Team Members: Ben Mitchell, Cody Bonds, Hudson McDiarmid and Rhett Caldwell
Industry Partner: National Safety Apparel - Arkansas
Industry Partner Contacts: Joey Roland, Plant Manager and Jennifer Gibson, Production Manager
National Safety Apparel is a company that creates quality clothing for those working in tough industries. Their Fort Smith, Arkansas, facility manufactures uniforms for the US Postal Service including various styles of shirts and pants. Our team is interested in increasing the production capacity and efficiency of the shirt assembly process by providing a decision support tool that will identify the bottlenecks and provide a resource allocation plan. By creating flow diagrams that represent how each style of shirt moves through the system and utilizing the SAM (standard average minute)/unit of each operation, we have identified the major bottlenecks in the system. We have created a Microsoft-Excel-based tool that will allow NSA-Arkansas to identify where to focus their improvement efforts and assist them in deciding which machines to upgrade based on the bottlenecks in the system. The tool will also take NSA-Arkansas's production goal and number of employees and output if the goal is feasible, the minimum number of employees necessary to accomplish the goal, and a suggested plan for the percent of time an employee should be assigned to each operation.
Using Simulation Analysis to Reduce Customer Sojourn Time in Tire and Battery Centers
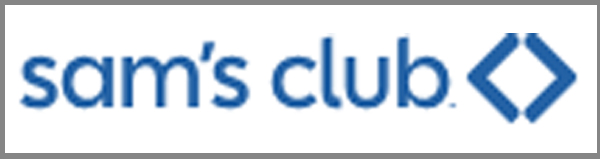
Student Team Leader: Jessica Creech
Other Student Team Members: Joshua Manson-Endeboh, Kaylee Harrison, Ronan McDonnell and Jacqueline Saldivar
Industry Partner: Sam's Club Tire & Battery Centers
Industry Partner Contacts: Mohamed Boudhoum: Senior Director of Tire & Battery Centers and Tim Gearhart: Project Manager III-Sam’s Club
Sam’s Club is a subsidiary of Walmart, Inc., that generates $80 billion annually using a membership-based business model. Customers pay an annual fee to be eligible to access the products and services that Sam’s Club offers including their grocery and retail shopping, health services, and tire and battery center. Sam’s Club offers customers in-person shopping at 600 locations across the United States; 582 of these locations offer multiple car repair services. These services include tire services, exchanging batteries, replacing windshield wipers, and restoring headlights. Sam’s Club has expressed concerns regarding the amount of time it takes to perform these services as well as the discrepancies in service times across locations. The goal of our project is to suggest improvements to the current system by analyzing the number of technicians performing each service given the number of bays available. To achieve our goal, we developed a simulation model in Arena using data that we collected via time studies. Our model allows technicians to float between vehicles to represent simultaneous technicians performing a service. The model allows us to find a new standard number of technicians for each service to reduce sojourn time without an increase in technician idle time.
Minimizing Changeover Time in Pet Food Production using Integer Programming
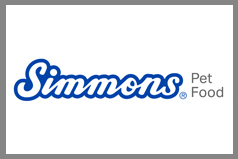
Student Team Leader: Ross Harper
Other Student Team Members: Halle Schneidewind, Griffin Langford, Danny Puente and Willow Franks
Industry Partner: Simmons Pet Food
Industry Partner Contacts: Ross Vandever, VP of Continuous Improvement; Cassie Warren, Continuous Improvement Manager and Jonathon Wilson, Project Manager in Pet Food Division
Founded in 1949, Simmons Foods, Inc., is a leading supplier of poultry, pet food, and animal nutrition products. Headquartered in Siloam Springs, Arkansas, Simmons Pet Food – a division of Simmons Foods, Inc. – is North America’s largest supplier of store brand wet pet food. Our focus is the scheduling process used to sequence the production of pet food batches. The current process is highly manual and lacks full visibility into accurate changeover times occurring between produced batches. Our team uses a predictive model fed by historical production data to better estimate these times. These estimates are used as a parameter in an optimization model with the objective of minimizing total changeover time during production. Using open-source optimization software and an optimization interface, our team expects to reduce the time to schedule production sequences by up to 25%. We also expect Simmons Pet Food to see reduced downtime and increased throughput as a result of lower changeover times.
Reducing the Cost of Inventory Counting with Improved Raw Material Storage
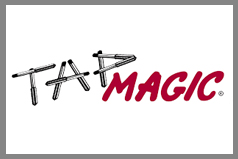
Student Team Leader: Whitney Hines
Other Student Team Members: Christopher Haywood, Jade Easter, Preston Boscamp and Rodolfo Bissot Stargardter
Industry Partner: Steco Corporation
Industry Partner Contacts: Ken Gaines, CEO and Louis Jaramillo, Operations Director
Steco Corporation is a small cutting fluids manufacturer located in Little Rock, Arkansas, that operates with only seven employees. Steco produces a line of cutting fluids called Tap Magic that helps to reduce friction on tools for various machine processes. Steco’s main concern involves their raw material inventory management. At the end of each month, limitations force Steco to perform a 100% blind count of all raw material inventory. This process takes 2-4 days to complete and results in a 91% inventory accuracy. We were tasked with providing a better inventory counting process that will minimize the time it takes to perform the count and increase overall inventory accuracy. We created a Microsoft Excel datasheet for our industry partners to use during the inventory count to track important information four months, the new data was used to calculate accuracy and identify items that were consistently inaccurate due to items being stored in multiple locations within the warehouse. We used the analysis to recommend that Steco invest in an RFID system, as well as use dedicated storage for consistently inaccurate items.
Developing A Monitoring Tool and Management Policy for University Research, Office and Conference Room Space
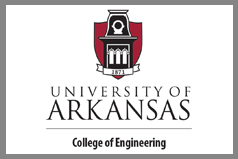
Student Team Leader: Tate Hasenclever
Other Student Team Members: Valerie Jackson, Foster Thompson, Kristoffer Olsen and Bowen Zhao
Industry Partner: University of Arkansas, College of Engineering
Industry Partner Contacts: Heather Nachtmann, PhD, Former Associate Dean for Research & Professor of Industrial Engineering and Kyle Cook, Facilities Manager
The University of Arkansas College of Engineering (CoE) adds social and economic value to the region, state, nation, and the world through engineering education and cutting-edge research in emerging technologies. With the wide variety of activities and recent growth, the CoE is beginning to see space constraining operations and is concerned about a future space shortage. To understand this problem, we began by performing external research, analyzing current data sources, and conducting stakeholder interviews. We learned that the space problem stems from the lack of a formal policy to set space standards, allocation procedures, and periodic review of space assignments. There also exists limited data insights or current metrics regarding space utilization. To improve space utilization for the CoE, our team developed a space management policy recommendation and a space management data monitoring tool. The recommended space policy outlines utilization metrics and targets by space type to inform CoE decision-makers on how to analyze and distribute space within the college. The data monitoring tool we created is a decision support dashboard that centralizes CoE space-related data and creates visualizations to gain insights and evaluate performance. Our hope is that this work will allow the CoE to make objective decisions as they adapt to future growth.
6th Annual Industrial Engineering Capstone Symposium
Arkansas Union Verizon Ballroom, University of Arkansas, Fayetteville
Wednesday, May 4, 2022
Balancing Workload by Optimizing the Assignment of Field Sales Proposals to Pricing Engineers
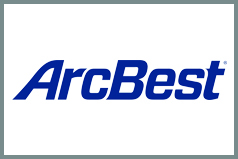
Student Project Manager: Luke Welch
Other Student Team Members: Aidan Massanelli, Andres Luna Orosco Amelunge, Hector Aguilar, and Harrison West
Industry Partner: ArcBest
Industry Partner Contacts: Erin Hills, Logistics Engineer; Drew Caple, Manger, Pricing and Supply Chain Analytics; and Alex Hoge, Senior Manager, Carrier Partnerships
ArcBest is a multibillion-dollar freight and logistics solutions provider located in Fort Smith, Arkansas. With over 40,000 capacity providers across North America, ArcBest solves complex supply chain problems for customers utilizing truckload, less-than-truckload, freight brokerage, and several other avenues of services. When a customer identifies a service that ArcBest can provide, a Field Sales Proposal is created and assigned to a pricing engineer. Once assigned, the Field Sales Proposal is labeled a Pricing Proposal Maintenance, or PPM. ArcBest has expressed concerns that pricing engineers sometimes experience a workload imbalance due to the PPM assignment process. The goal of our project is to improve the process of assigning Field Sales Proposals to pricing engineers. By utilizing PPM data provided by ArcBest, our team performed a regression test on different types of PPMs to provide an estimated mean service time for each type. Then, we developed a Java application that assigns batches of PPMs to engineers in a way that minimizes the difference of workload between engineers. This optimization approach is expected to reduce the workload imbalance and result in a more even utilization of engineers and faster service times for PPMs.
Reducing Patient Transportation Times through Improved Staff Scheduling using Simulation
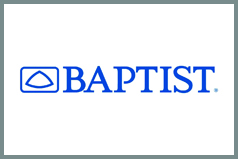
Student Project Manager: Olivia Gammill
Other Student Team Members: Taylor Knabe, Jennifer Sanchez, Andrew Hume, and Patrick Gonzalez
Industry Partner: Baptist Memorial Hospital-Memphis
Industry Partner Contacts: Katie Parker, Regional Director for Performance Improvement; and Jared Moses, Administrative Director at Baptist Memphis
Baptist Memorial Healthcare Corporation is a network of hospitals located throughout the central-south of the United States that offers patient treatment and medical care with various specialties. Our interest is in patient transportation at Baptist’s flagship hospital in Memphis, Tennessee. Transportation refers to team members, called transporters, who pick up and move patients between different areas of the hospital. Baptist is concerned with excessive patient transportation times. We identified that the Memphis hospital is falling short of Baptist’s 35-minute transportation goal. On average, transportation time takes 51.6 minutes on weekdays and 44.6 minutes on weekends. We determined that 82% of the total transportation time is spent waiting for the transporter to be ready. These long waiting times suggest a staffing issue. To explore this issue, we built a detailed discrete-event simulation model of the transportation process. We used this model to experiment with different staffing strategies. By more effectively utilizing the staff Baptist already has, we were able to decrease the average transportation time to 40 minutes where 55% of transports were meeting the goal of 35 minutes or less. By increasing the number of staff to 40, we found that the average transportation time decreased to 31 minutes and the percentage of transports completed in 35 minutes or less increased to 74%.
Improving the Scheduling of Protection Services Staff by Creating a Microsoft Excel Application and Exploring Shiftboard’s Full Functionality
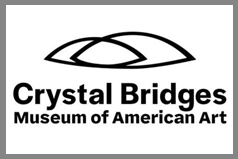
Student Project Manager: Natalia Sandhu
Other Student Team Members: Camila Schrader, Itza Della-Sera, Sarah Bollinger, and Esteban Siles
Industry Partner: Crystal Bridges Museum of American Art
Industry Partner Contacts: Kash Logan, Director of Protection Services; Brent Pettingill, Protection Services Manager; and Michelle Mittiga, Administrative Assistant
Crystal Bridges Museum of American Art is a non-profit charitable organization that celebrates the American spirit in a place that unites the power of art with the beauty of nature. Our interest in Crystal Bridges is their Protection Services department, specifically the scheduling of Protection Services staff. This department provides a safe environment for employees and guests while also protecting the museum’s art. Currently, Crystal Bridges has concerns due to the inefficiency of creating schedules and the inefficient use of Shiftboard. Shiftboard is the software the Protection Services department uses to distribute the completed schedules to the employees. The administrative assistant responsible for creating the schedules takes approximately 3 hours per week to create the schedules, with 33% of time spent manually transferring the completed schedules into Shiftboard. To reduce this time, our team created two Microsoft Excel tools that eliminate Shiftboard usage. For the first tool, every employee is allowed access to one worksheet, accessed only with a username and password, that has the corresponding employee’s schedule for the upcoming two weeks. With the second tool, the administrative assistant clicks a button to automatically send the employee’s schedules to their individual email. Additionally, we found ways to improve the current use of Shiftboard. We mastered details of Shiftboard’s functionality, converted that functionality to Crystal Bridges, provided documentation of Shiftboard’s relevant tools, and taught the administrative assistant how to properly use the features. We use simulation to compare the use of the new Excel tools to the current process, and we use optimization to offer general guidance on fair scheduling practices.
Decision Making for Downstream Pace in the Event of Upstream Delays
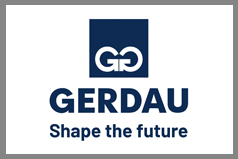
Student Project Manager: Neil Balasekaran
Other Student Team Members: Peyton Dunman, Noah Layton, Bailee Miller, and Maddie Pearl
Industry Partner: Gerdau
Industry Partner Contacts: Nicholas Boerjan, Melt Shop Department Manager; Clinton Johnson, Organizational Development Specialist; and Heather Christensen, Process Engineer
Gerdau is a steel manufacturing company located in Fort Smith, Arkansas, that specializes in the production of steel billets. Our interest is in the pace at which the six-step steel making process of the melt shop is operating. When a delay occurs at an upstream process, the downstream caster pace is negatively impacted. Through stakeholder interviews, our team concluded improper pacing of the melt shop, due to these unpredictable delays, leads to a reduction in the caster’s Tons Per Hour (TPH), a throughput key performance indicator for the melt shop. Our goal was to create a tool that aids in decision making for setting caster pace (TPH) upon different melt shop delays and improves data collection for future delay analysis. To accomplish this goal, we designed a tool that allows for visibility of station progress. This system requires a data collection component that allows each station to record their progress, a data analysis element for the floor manager to better predict an operating TPH, a monitoring system for the floor manager to see the progress within each station, and a user interface that is easy for all operators to use.
Developing a Consolidated Toxicological Database to Perform Chemical Risk Analysis and Predictions
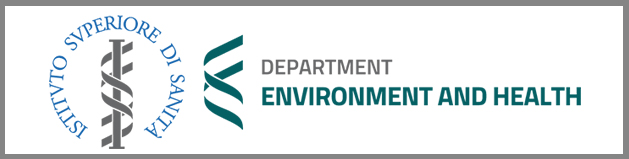
Student Project Manager: Quinn Salverson
Other Student Team Members: Andrew Banks, Colin Connelly, Kaitlyn Frey, and Isha Rajaram
Industry Partner: The Instituto Superiore Di Sanitá - Department of Environment and Health
Industry Partner Contacts: Dr. Olga Tcheremenskaia - Principal Investigator; Dr. Chiara Battistelli - Principal Investigator; Dr. Cecilia Bossa - Principal Investigator; and Cristina Parenti - Sinergi llc, Professional Affiliate
The Instituto Superiore di Sanità (ISS) acts as the main center for research and advice on public health in Italy. The Department of Environment and Health of ISS aims to reduce environmental and social risk factors through risk assessments that include the development of predictive toxicology models for speeding up the toxicological assessment of chemicals and, at the same time, for reduction of animal testing. The predictive toxicology approaches share the need of highly structured and standardized data as a starting point. ISS currently utilizes five separate data sources for carcinogenicity and mutagenicity called the ISSTOX to combat animal testing, with each dataset defining different chemical attributes and testing information for analysis. A 2020-2021 industrial engineering capstone team at the University of Arkansas initially sought to improve the interoperability of the ISSTOX data by designing a relational database model and proposing a user-interface that allows for chemical visualization and analysis tools. The primary goals of our project are to design and implement a simplified version of the relational database model in Microsoft Access and to design and implement a system allowing for data integration, visualization, and analysis. The finalized Microsoft Access relational database will allow public use of toxicological data to perform chemical risk and predictive analysis.
Maximizing Utilization of Intermodal Local Tractors through Driver Reassignment
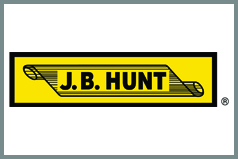
Student Project Manager: Coleman Warren
Other Student Team Members: Faris Balbaid, Eric Gerstein, William Fletcher Rosenbleeth, and Hayden VanLaningham
Industry Partner: J.B. Hunt Transport Services, Inc.
Industry Partner Contacts: Sara Pierson, Logistics Engineer II; and Jordan Sonnentag, Senior Logistics Engineer
J.B. Hunt Transport Services, Inc., is a Fortune 500 company headquartered in Lowell, Arkansas, that provides a variety of freight transportation services for customers in North America. J.B. Hunt Intermodal (JBI) delivers freight to consumers using a combination of truck and rail delivery systems. JBI seeks to minimize cost per mile and maximize tractor utilization on regional and local tractors for the life of the tractor. Driver reassignments or tractor transfers between fleets occur to balance tractor utilization but are infrequent and lack a decision-making process. Tractor utilization is not closely monitored even though large costs are incurred from both opportunity costs for underutilization and fees for overutilization of JBI tractors. We created a weekly monitoring system that identifies mis-utilized tractors and displays these tractors on Power BI. We also created a driver reassignment recommendation tool that considers tractor utilization and driver productivity to provide an assignment of drivers to tractors within a fleet to balance tractor utilization. We established a database structure for JBI to utilize when inputting data into our system to reassign drivers within fleets and tractors between fleets. Finally, we documented the process of evaluating the success of a transfer and studied the effects of our tool on a simulated model of a JBI local fleet.
Data Synthesis, Storage, and Visualization for Near Real-Time Analysis of Final Mile Delivery Routes
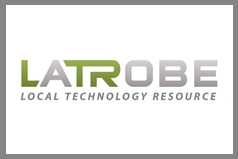
Student Project Manager: Spencer Loper
Other Student Team Members: Henry Ward, John Santine, Luke Rafie, and Cody Parrish
Industry Partner: LATROBE LLC
Industry Partner Contacts: Latanyua Robinson, President; and James Robinson, Vice-President
Founded in 2010, LATROBE LLC is a consulting firm operating transactional businesses to provide livable wage job opportunities in manufacturing and transportation. The Mission of LATROBE LLC is to advocate for Economic Empowerment thru Education + work Experience + Entrepreneurship. Since 2019, LATROBE has entered a Delivery Service Partnership (DSP) with Amazon, acting as a subcontractor for final mile delivery. In completing their delivery routes, LATROBE receives data from multiple, independent applications that Amazon provides. Currently, no process or mechanism exists for LATROBE to synthesize and leverage their data in making business decisions. The goal of our project is to design a system to turn LATROBE’s multiple data sources into meaningful analytics and visualizations. To accomplish this goal, we designed a system composed of three subsystems: extract, transform, and load (ETL), data storage, and data visualization. In designing this system, we provided LATROBE with two solutions: our original proof-of-concept tool that could be implemented immediately, and our proposed future work to add more capabilities and automation if LATROBE wants to invest more in this area.
Improving the Usability and Accuracy of a Category Sales Forecasting Tool
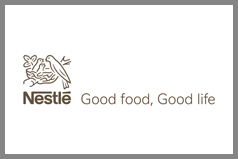
Student Project Manager: Chloe Kordsmeier
Other Student Team Members: Grant Glover, Collin Snieski, Caleb Gonzales, and Carolina Virreira
Industry Partner: Nestlé
Industry Partner Contacts: Edosa Aibangbee, Customer Account Manager; Raegon Barnes, Director Category Management; Ian Rippl, Customer Account Analyst; and James Gairhan, Category Sales Analyst
Nestlé is the world’s largest food and beverage company, and Nestlé USA is a branch of the company that provides a variety of products to retailers across the United States. Nestlé USA’s office in Rogers, Arkansas, is focused on Nestle’s relationship with Walmart. Nestlé USA has three category advisorships with Walmart: Baking, Chilled Creamers, and Frozen Meals; Walmart trusts Nestlé to offer advice on product placement and quantity in those categories. Our project is focused on improving the forecasting tool created by a 2020-2021 University of Arkansas industrial engineering capstone team. Currently, Nestlé USA uses the previous year’s sales data as the prediction for the current year’s sales instead of using the tool created by the 2020-2021 team. Nestlé USA asked us to improve the user interface of the tool and to extend the forecast horizon while maintaining a low mean absolute percent error. To improve the forecasting horizon of the previous tool, we weighted the 2020 sales data to adjust for the impact of the COVID-19 pandemic. To address the tool’s user-friendliness, we altered the R code to allow the user to choose their file and automatically count the number of weeks for historical data, eliminating the need for the user to have to alter the code.
Balancing Hospitalist Workload by Optimizing Patient Assignment using Linear Programming
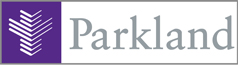
Student Project Manager: Brandon Jerome
Other Student Team Members: Jaiden Ellerbee, Ryan Kraichely, Terrance Martin, and Camille Sockwell
Industry Partner: Parkland Health
Industry Partner Contacts: Nina Drolc, Executive Nursing Leadership, Senior Analyst, Database; and Nainesh Shah, M.D., Deputy Medical Information Officer
As one of the largest public hospital systems in the United States, Parkland Hospital serves Dallas County, Texas. Patients with medical diagnosis are admitted and assigned to a hospitalist, a physician trained in hospital medicine tasked with day-to-day care of medicine inpatients. Parkland Hospital is concerned with the amount of time hospitalists spend each morning assigning patients to hospitalist teams, and the resulting workload imbalance among hospitalists. To automate the assignment process, we created a decision support tool that utilizes Microsoft Excel and Visual Basic for Applications to read in patient data, clean and format the data, calculate severity scores per patient, and output a file that is then read in by a Python script. The script runs an integer program built to optimize patient assignments. Our model outputs proposed patient assignments with the objective of balancing workload among hospitalists while also considering the unit location preferences of hospitalists. In addition to optimizing workload balance, our tool will reduce the time required to assign patients to hospitalists by 75-85%.
Reducing Bone, Liver, and Lung Biopsy Turnaround Times for Potential Oncology Patients by Standardizing System Processes
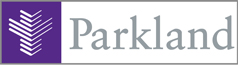
Student Project Manager: Shantal Sarmiento
Other Student Team Members: Tayden Barretto, Stephen Branscum, and Paola H. Franco
Industry Partner: Parkland Health
Industry Partner Contacts: Nina Drolc, Executive Nursing Leadership, Senior Analyst, Database; Jenni Burnes, Vice President, Ancillary Services; and Robyn Cobb, Program Manager
Parkland Health is one of the largest public hospitals in the U.S. Opened in 1894, Parkland is a safety net provider that serves uninsured, underinsured, and lower-income patients. Parkland offers multiple services within the hospital, but we are specifically interested in the interaction of medical imaging (radiology) and cancer care (oncology). Our goal is to reduce biopsy turnaround times of potential oncology patients to less than 14 days from biopsy order to biopsy appointment completed. We conducted analysis on different data sets provided by Parkland to determine the system's steps and how long it takes a patient to move between each major stage in the process. We identified problems related to data recording, lack of standardized processes, and appointments scheduled without patients' awareness. We wrote VBA code to reorganize the data for easier analysis, and we performed a linear regression to show the relationship between time to complete the process and day, month, and year. In addition, we developed a simulation model to support our recommendations for system standardization, to reduce turnaround times, and to improve the overall patient experience.
Improving the Centralized Lending Processes for Increased Throughput using Targeted Human-Centered Solutions
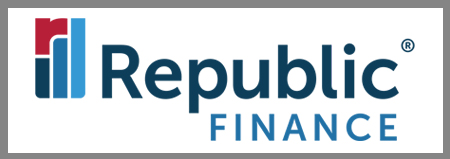
Student Project Manager: Luke Weiner
Other Student Team Members: Cleondra Cooks, Anna Lee, Jaira Porter, and Aidan Grygar
Industry Partner: Republic Finance, LLC
Industry Partner Contacts: Sherwin Poormand, Head of Loss Mitigation Strategy; and Savensky Drakeford, Head of Central Collections
Founded in 1952, Republic Finance specializes in providing a variety of consumer loans, flexible lending options, and incomparable customer service. They have assisted customers in meeting their personal finance goals for nearly 70 years. With branch offices located in Alabama, Georgia, Kentucky, Louisiana, Mississippi, Missouri, South Carolina, Tennessee, Texas, and Virginia, Republic Finance is proud to serve more than 300,000 customers in over 250 communities throughout the United States. Republic Finance provides flexible lending options to customers through both localized branches and a Central Lending Unit (CLU). While branches work directly with customers located within a specified geographical footprint, the CLU operates over-the-phone across the entire Republic Finance customer base, including those customers who are not located near a branch. We worked with the CLU to identify and provide solutions for several problem areas within their remote lending process. Several opportunities were identified within the steps of the process including initial solicitation, agent-to-customer interaction, receiving customer documents, and contract execution. Focusing on mutually exclusive and collectively exhaustive solutions to address all parts of the loan-by-phone process, our team generated five solutions with different targeted improvements. To improve initial solicitation and contract execution, a modified agent script and online appointment booking system were designed. A digital collateral list and document checklist were created to enhance the agent-to-customer interaction model. Lastly, using random forest modeling, primary factors with the highest correlation to a customer filling an application after solicitation were identified and we recommended a possible path forward to prioritize customers with a higher probability of applying for a loan.
Improving the Sustainability and Reducing Costs of Inbound Loads using Lane Consolidation
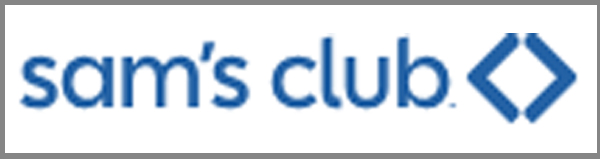
Student Project Manager: Emma Regier
Other Student Team Members: Lucas Hicks, Emily Eskens, Jacob Reich, and Austin Wood
Industry Partner: Sam's Club
Industry Partner Contacts: Abhishek Devadiga, Manager II, Supply Chain Management; and Ben Grunow, Sr. Manager Outbound Transportation
Sam’s Club is a retail warehouse club managed in conjunction with Walmart that operates out of large facilities and charges their club members annual fees to shop at their stores. Sam’s Club’s distinctive business model allows customers to buy items in bulk. Our system of interest is their inbound transportation network that is responsible for transporting products from vendors or import distribution centers to center points, Sam’s distribution centers, or fulfillment centers. Our initial goal was to investigate the use of heavy haul permits to reduce empty miles, but we found that divisible loads cannot legally be considered for heavy haul. We then pivoted our focus to a load consolidation effort. Sam’s Club asked us to design and develop a decision-support tool to evaluate the cost savings and sustainability impacts associated with load consolidation opportunities. To accomplish this, we created a tool through Power BI that identifies lanes that are underutilized and provides potential savings and sustainability improvements if consolidation efforts were to be implemented. Once connected to Sam’s Club’s live data source, this tool will be able to track and identify consolidation opportunities within lanes for the inbound transportation network.
5th Annual Industrial Engineering Capstone Symposium
Reducing Empty Costs of Relocube Repositioning through Forecasting and Optimization
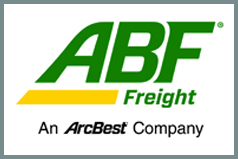
Student Project Manager: Laura Acosta
Other Student Team Members: Tate Thornley, Joseph Cummings, IV, Kate Burrows, and Xander Smith
Industry Partner: ABF Freight
Industry Partner Contacts: Jacob Huffstetler, Senior Financial Analyst; and Kirby Clark, Director, Financial Planning & Analysis
ABF Freight provides less than truckload shipping using a nationwide network of 242 service centers. ABF Freight offers U-Pack, a self-pack moving service that uses containers called ReloCubes to store customer belongings while in transit to the client’s new home. Because U-Pack operates from a nationwide network of service centers, it is common for ABF Freight to reposition ReloCube containers from one service center to another to meet customer demand. The goal of our project is to reduce the empty costs associated with ReloCube repositioning. We created an inventory model to track the movement and storage of ReloCubes throughout the service center network. Based on the inventory model, we created an optimization model that prescribes when, from where, and to where ReloCubes should be repositioned. The inputs to our optimization model include forecasted ReloCube demand based on data from 2016-2020. With the use of our models, ABF Freight now has the ability to reduce the distance and costs associated with ReloCube repositioning.
Improving the Offer Creation Process by Standardizing ArcBest’s Bid Model
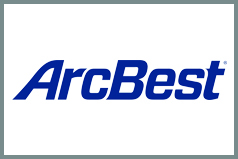
Student Project Manager: Ben Barron
Other Student Team Members: Jackson Marshall, Ben Baser, Blake Parrish, and Joseph Ellis
Industry Partner: ArcBest Corporation
Industry Partner Contacts: Trenton Cason, Lead Logistics Engineer - Yield Strategy and Alex Hoge, Pricing and Supply Chain Manager
ArcBest is an integrated logistics provider headquartered in Fort Smith, Arkansas. They are focused on providing the best customer experience possible with seamless access to a broad suite of logistics capabilities, including truckload, LTL, ocean and air, ground expedite, managed transportation, and warehousing. Our system of interest falls within the yield division where the goal is to create and return pricing bids to potential customers. ArcBest’s yield division currently lacks standardization in bid formats when bid offers are created and transferred to the marketing and sales teams which causes redundancy and extra time for employees across multiple areas. Our system improvement efforts are aimed at automating and standardizing the creation of offers within the bid process. We created a tool in Microsoft Excel using Visual Basic Userforms. The tool is designed to minimize the work required to create an offer. It is impossible to create a tool for every situation a Pricing Engineer will encounter, so we aimed at building an extensible tool that can be the foundation for future add-ons. To estimate the potential impact of our tool on bid processing, we created a simulation model. Based on historical data on 10,000 bids, we simulated bid processing with features such as bid type, sales region, and bid market. Our analysis shows that implementing our solution should yield a 1.9% improvement in engineer utilization which will allow for more bids to be processed without hiring more engineers. Our analysis also shows that our solution will decrease the amount of time required to process a bid by 5% or more than 38,000 hours annually.
Reducing Emergency Department Wait Time by Improving Nurse Scheduling using Simulation
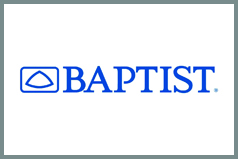
Student Project Manager: Matt Nixon
Other Student Team Members: Junwoo Chang, Jacob Kinney, Matt Murry, and Hawkin Starke
Industry Partner: Baptist Memorial Healthcare
Industry Partner Contacts: Katie Parker, Regional Director of Performance Improvement
Baptist Memorial Healthcare is a large healthcare organization operating in the southeastern United States. Their flagship hospital, located in Memphis, TN, treats more than 65,000 patients in its Emergency Department (ED) every year. The ED is comprised of two main processes: the front-end process includes patient arrival, registration, and initial triage (condition assessment); the back-end process includes patient treatment. Extended patient wait times are the main concern of our industry partner. Our project focuses on the staffing needs of the front-end process with the goal of reducing patient wait time. By reducing patient wait time, the ED can also reduce patients leaving without being seen by a provider (LWBS). To better understand this process, we created a simulation model that mimics the flow of patients into and through the ED. The simulation models arrivals to the ED as a function of time of year, day of week, and time of day. We explore the levels of nursing staff required within the front-end of the ED to provide a high-quality patient experience while maintaining high utilization of nursing staff.
Reducing Length of Stay Overages by Improving Hospitalist’s Rounding Paths and Patient Assignments
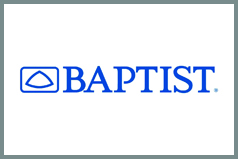
Student Project Manager: Lauren Law
Other Student Team Members: Adam Corral, Courtney Johnston, Dania Quintero, and Owen Stuckey
Industry Partner: Baptist Memorial Healthcare
Industry Partner Contacts: Katie Parker, Regional Director of Performance Improvement
Our industry partner is Baptist Memorial Health Care. Our project involves Baptist’s Memphis hospital, the largest hospital in the network. As they do for all hospitals, insurance companies cover treatment for patients at the Memphis hospital a certain number of days based on diagnosis. This number of days is called Observed to Expected (O-E). Baptist is concerned that patient Length of Stay (LOS) too often exceeds the O-E and asked us to investigate potential causes and solutions involving hospitalists. Hospitalists are doctors who make daily rounds to a set of patients to track their progress and ultimately discharge them. We found that hospitalists spend a lot of their day traveling between patient rooms, because patient room location is not considered in the assignment of patients to hospitalists. They also make frequent return trips to their offices in between patient visits to input orders and make notes. This excess travel implies less time to spend with patients. Our goal is to reduce unnecessary travel time for hospitalists, giving them more time for patients, thus leading to reduced LOS. We achieve this goal by improving patient to hospitalist assignment policies and providing workstations closer to patient rooms.
Predicting Transitions of Steel in the Molten Scrap Metal Casting Process
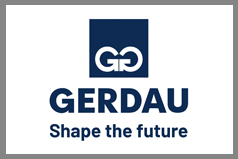
Student Project Manager: Esteban Lopez
Other Student Team Members: Emily Feuerborn, Linden Van Hoose, and Logan Rodriguez
Industry Partner: Gerdau
Industry Partner Contacts: Clinton Johnson, Management Systems Facilitator; Eric Springs, QA & Metallurgy Manager; Alan Doss, Quality Labs Routine Facilitator; and Caleb Collins, Caster Specialist
Gerdau is a steel manufacturing company that produces long steel and special steel products. For this project, we worked with Gerdau’s mill located in Fort Smith, Arkansas. Our system of interest is the transition phase of their continuous casting process. The transition between two customer orders produces a mix of steel. This transition metal does not satisfy the chemistry composition requirements for either order and therefore must be cut out and scrapped. The transition technicians manually estimate the amount of transition metal based on rough guidelines and experience. This approach leads to unacceptable estimation errors. When the transition is overestimated, quality steel meant for the customer is cut, and good steel is wasted. Underestimating does not allow the full transition to be cut out from the quality steel before it moves on to the next phase of the manufacturing process. We improved the estimation process by creating a multiple linear regression model and a random forest model. We implemented these models in a spreadsheet tool that also automates the transition technicians’ guidelines for their current approach.
Reducing Production Costs by Optimizing the Assignment of Parts to Machines using Cost-Benefit Analysis and Monte Carlo Simulation
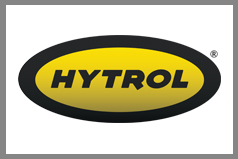
Student Project Manager: Matthew Walters
Other Student Team Members: Cameron Blann, Christofel Enslin, Alyssa McKnight, and Jasia Porchay
Industry Partner: Hytrol Conveyor Company, Inc.
Industry Partner Contacts: Felton Barlow, Manufacturing Engineer; and Ken Nickerson, Industrial Engineering Manager
Hytrol Conveyor Company, Inc., is an engineered to order (ETO) manufacturing company that produces conveyors for customers such as UPS, FedEx, and Amazon. Our project’s system of interest is the laser cutting and punching operations within Hytrol's brown and silver fabrication cells. Our initial goal was to reduce the amount of scrap produced from these two cells. Using Pareto analysis, we discovered a shift in the main cause of scrap from the laser cutter in 2018 to the punch in 2020. From 2018 to 2020, the amount of scrap produced from laser cutters decreased by 50% while the amount of scrap from punches increased by 30%. This shift was a result of Hytrol actively moving the production of their parts from the laser cutter to the punch in an attempt to standardize production. Because Hytrol continues to strive to standardize their operations, we switched our focus from scrap to production costs. We identified a need for a cost-benefit analysis tool to assist Hytrol in determining if switching the production of a specific part from the laser to the punch would be cost effective. A Monte Carlo simulation is used within the tool to account for the uncertainty of certain parameters used to calculate expected net present value, payback period, and return on investment.
Facilitating Chemical Risk Analysis by Organizing Toxicological Databases with a Flexible Open Platform
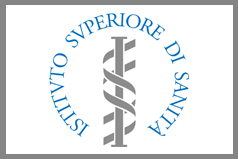
Student Project Manager: Conner Waybright
Other Student Team Members: Ryan Brim, Yok Lin Ong, Brandon Ward, and William Warner
Industry Partner: Istituto Superiore di Sanità
Industry Partner Contacts: Cristina Parenti - Freelance Consultant; Olga Tcheremenskaia, PhD – Principal Investigator; Cecilia Bossa, PhD – Principal Investigator; and Chiara Laura Battistelli, PhD – Principal Investigator
Istituto Superiore di Sanità (ISS), or the National Health Institute of Italy, is an organization whose mission is to guide public health policy based on scientific research. Demand for a shift away from chemical testing on animals has led ISS to take steps towards analyzing chemical toxicity with alternative computational methods using existing data. ISS has gathered and stored a collection of toxicological data in five data sources called ISSTOX. The purpose of our project is to further develop ISSTOX by increasing the interoperability of the data. Our goal is to facilitate the creation of a public toxicological data storage and analysis system using the ISSTOX data. To accomplish this goal, we must design a system that facilitates chemical risk assessment using legacy data. Integrating the data sources of ISSTOX requires data cleaning and standardization before consolidating the five data sources into a single, chemical relational database. This process requires designing a data model sufficient to store and retrieve the ISSTOX data. This system also includes a user-interface designed to interact with the database, allowing users to conduct useful toxicity analyses. Finally, this system is designed for a publicly available, online setting to increase accessibility to quality toxicity data.
Balancing Inbound and Outbound Flow of Trailers by Predicting Customer Compliance and Seasonality
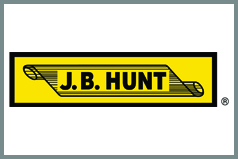
Student Project Manager: Parker Tankersley
Other Student Team Members: Grayson Lee, Remy Kirk, Jacob Underhill, and Alyssa Roth
Industry Partner: J.B. Hunt 360 Box Engineering Team
Industry Partner Contacts: Matt Turner, Logistics Engineer I and Elisa Daniel, Logistics Engineer II
J.B. Hunt is a transportation and logistics company that moves customer freight throughout North America by both truck and railway. We worked with J.B. Hunt’s 360 Box service that offers drop and hook trailer shipping options. 360 Box utilizes J.B. Hunt’s online marketplace to pair third-party and J.B. Hunt trucks to trailers for transport. It is crucial for 360 Box to keep trailer flow in and out of customer markets balanced to avoid empty miles and off-route travel. With our partners within the 360 Box Engineering Team, we identified lack of compliance with expected load volumes and seasonality as the main challenges to maintaining balance. We addressed these challenges by creating a random forest regression model that predicts customer compliance as a function of information contained within award contracts and by creating a 12-month volume forecasting tool using Holt-Winters’ method to account for seasonality. These tools will allow 360 Box to make confident, data-driven trailer balancing decisions. Based on trailer balance situations explored in historical data, our team estimates a 27% reduction the mean absolute percent error in compliance prediction when using our random forest regression model, with additional balancing insights drawn from our seasonality tool.
Improving Driver Integration across Dedicated Contract Services Accounts using Resource Identification and Capacity Planning
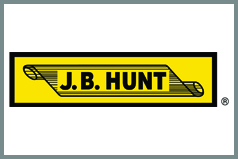
Student Project Manager: Brianna Bert
Other Student Team Members: Jose Beltran, Gabriel Figueroa, Sam Griffin, and Emily Rodriguez
Industry Partner: J.B. Hunt Transport Services, Inc.
Industry Partner Contacts: Zach Evans, Senior Logistics Engineer; Graham Nelson, Logistics Engineer III; and Marc Alley, Senior Director of Engineering and Technology
J.B. Hunt Transport Services, Inc., is a transportation and logistics company that serves clients through their five business units: Intermodal, Integrated Contract Services, Truckload, Final Mile, and Dedicated Contract Services (DCS). We partnered with J.B. Hunt’s DCS business unit to investigate ways that we can help increase driver utilization in their western region. DCS Drivers on average work approximately 55 hours/week. Because of fluctuations in account needs due to seasonality and other factors, drivers often are not being utilized to their full potential. Currently, DCS account managers work to integrate drivers across DCS accounts by contacting other account managers to identify available loads when their account is overstaffed or available drivers when their account is understaffed. Standardizing the process for account managers to identify available drivers and loads in other accounts would result in increased driver utilization. To eliminate the need for account managers to independently seek out opportunities for their drivers, we developed a database-driven tool that has two main features: driver availability identification and utilization tracking. This tool aims to identify drivers that meet an account’s specifications while also prioritizing drivers with lower utilization and closer proximity to the account in need of drivers. The database tool will expand the network of available drivers and loads to maximize driver utilization and reduce net driver needs of JB Hunt. The tool will also continuously track utilization and forecast driver needs for all dedicated accounts.
Improving Multi-Stop Truckload Pricing using Random Forest Regression
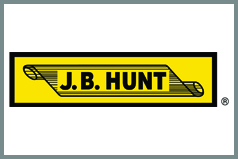
Student Project Manager: Madeline Suellentrop
Other Student Team Members: Garret Clark, Chase Cottrell, Nicholas Jaco, Jaclyn Walls
Industry Partner: J.B. Hunt Transport Services, Inc.
Industry Partner Contacts: Doug Mettenburg, Becca Luetjen and Kyle Kraichely
J.B. Hunt Transport Services, Inc. is a Fortune 400 company that provides transportation and logistics solutions using trucks and trains to move freight across North America. Within J.B. Hunt, Integrated Capacity Solutions (ICS) conducts third-party logistics by pairing non-contract customers with third-party carriers. ICS uses their own software, ETP, to predict the cost of multi-stop truckloads. Multi-stop truckloads combine Less-Than-Truckload customer orders with a single origin and multiple destinations. The current process used to estimate the cost of a multi-stop shipment has not been evaluated in approximately ten years; therefore, our goal is to improve multi-stop truckload cost prediction. To improve the accuracy of cost estimation, we applied a machine learning technique called random forest regression. Under J.B. Hunt’s current approach, the Mean Absolute Percent Error (MAPE) of multi-stop cost estimates is 20.1%, but with the applied random forest regression model, the MAPE is now 12.5%. With improved estimation accuracy, J.B. Hunt can achieve higher customer satisfaction leading to revenue retention and growth.
Forecasting Category Sales using Data Mining and Predictive Analytics
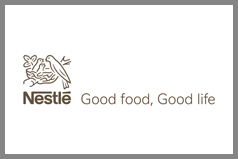
Student Project Manager: Amie Beckwith
Other Student Team Members: Suelly Samudio Ortega, Joseph Goodman, Sebastian Alborta Pereyra
Industry Partner: Nestlé USA
Industry Partner Contacts: Raegon Barnes, Director Category Management; Emily Power, Sr. Analyst Category Management; and Edosa Aibangbee, Manager Category Management
Nestlé USA is a segment of Nestlé, the world’s largest food and beverage company. Nestlé USA provides a variety of food and beverage products to retailers across the United States. Nestlé USA’s office in Rogers, Arkansas, focuses on its Walmart and Sam’s Club operations. Nestlé USA has category advisorships with Walmart, meaning that they have been chosen by Walmart to offer professional advice on product assortment for these categories. Our project is focused on improving Nestlé USA’s sales forecasting system for its category advisorships. Currently, Nestlé USA simply uses last year’s sales as an estimate for this year’s sales. This system has proven to be inefficient as it is entirely backwards looking. To improve Nestlé USA’s forecasting system, we implemented ARIMA forecasting models using R. These models incorporate binary holiday variables and detect growth and decline over time. Our analysis shows that these models offer a clear improvement from the current system, especially when using short forecasting horizons. To add ease to the forecasting process, we also created a user interface for our forecasting models using R Shiny. This interface allows the user to make custom selections and press a button to output the desired forecast.
Automated Pairing of Clients to Job Opportunities based on a Quantitative Assessment of Skills and Abilities
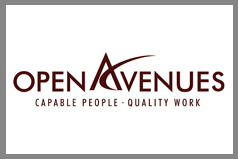
Student Project Manager: Lawson Porter
Other Student Team Members: Patrick Dougherty, Andrew Powers, and Hatem Alsayed
Industry Partner: Open Avenues
Industry Partner Contacts: Jeff Hairston, Training Center and Operations Manager
We partnered with Open Avenues, a local non-profit organization that helps people with disabilities (clients) attain vocational goals. Open Avenues offers a Community Employment service which seeks to place clients into job opportunities in the local community; however, the current process has challenges with matching clients to jobs that are appropriate for their abilities. To improve this service, our team developed an automated abilities-based tool which allows Open Avenues staff to match clients to jobs from a database of over 800 occupations. The tool also allows for the input and storage of pertinent client information, including the results of the abilities assessment clients complete upon arrival to Open Avenues. This tool will equip Open Avenues with the client-specific recommendations needed to place clients into roles where they can succeed both personally and professionally.
Improving Course and Classroom Scheduling Policies through Correlation Analysis and Forecasting
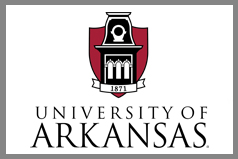
Student Project Manager: Michael Rechtin
Other Student Team Members: Alyssa Bobalik, Olivia Hope, and Jacob Pannell
Industry Partner: The Office of the Provost at the University of Arkansas
Industry Partner Contacts: Gary Gunderman, Executive Director of Institutional Research and Assessment and Colleen M. Briney, Vice Provost for Planning
This project aims to assist the Office of the Provost at the University of Arkansas by analyzing and improving the course and classroom scheduling process. The responsibilities of the Office of the Provost include overseeing academic policies, budgetary affairs, and the three parties that control this scheduling process: the colleges, their departments, and the Office of the Registrar. The scheduling process starts by exporting the previous year’s semester schedule. Next, all course details are edited and approved by all three parties before courses are assigned classrooms. Later, if a student wishes to enroll in or drop a course after enrollment, an override is used. Within the scheduling process, we focus on increasing classroom utilization, forecasting core course enrollment, and improving the override sub-process. We evaluate the relationship between classroom utilization and the characteristics of a room using correlation analysis. Additionally, we analyze classroom utilization by time of day and the day of the week. Next, we forecast core course enrollment using ARIMA. Lastly, we recommend improvements to the Department of Industrial Engineering’s override process.
Increasing Violation Capture Rates by Improving Parking Control Routing and Scheduling
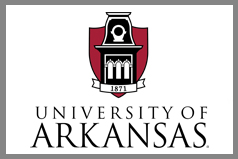
Student Project Manager: Warren Lewis
Other Student Team Members: Sam McKinney, Kyle Sprang, and P. Marshal Smith
Industry Partner: University of Arkansas Transit and Parking Department
Industry Partner Contacts: Gary K. Smith, Director, Transit and Parking and Andy Gilbride, Project/Program Manager
The University of Arkansas Transit and Parking Department serves the university community by providing transit and parking options to staff, students, faculty, and visitors. It is important to the department’s mission that fair parking opportunities are provided, so vehicles in violation of regulations should be identified and issued a citation. The department is concerned that a number of parking violations are missed by their Parking Enforcement staff. The department’s enforcement is made up of Parking Control Officers (PCOs) who are in charge of patrolling the university parking lots and garages in order to issue citations for any identified parking violations. We worked with the department to increase the effectiveness of the parking patrol process. We used data analysis, system engineering, and optimization techniques to provide the department with updated PCO routing and scheduling processes that improve the system’s parking violation capture rate by reducing travel time and improving patrol coverage.
4th Annual Industrial Engineering Capstone Symposium
With the end-of-year capstone symposium cancelled this year due to the pandemic, the awards were made based on the entirety of the work and efforts of the teams throughout the two semester course.
3rd Annual Industrial Engineering Capstone Symposium
Improving Capital Investment Decisions by Increasing the Fidelity of Return on Capital Employed
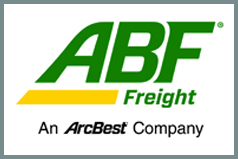
Team Members: Kyle Kraichely, Project Manager; Austin Knabe, Sydney Motes, Theo Munoz, and Josselyn Terrazas
Industry Partner: ABF Freight®
Industry Partner Contacts: Kirby Clark - Sr. Manager of Financial Analysis, Engineering & Pricing and Jacob Huffstetler - Lead Financial Analyst
ABF Freight is one of the largest LTL motor companies and provides service to 98%
of U.S. cities in all 50 states. ABF Freight is interested in determining the quality
of their capital equipment investments by estimating how these investments will perform
over time in terms of expenses incurred and the effect on the overall financial performance
of the company. The company measures the value of an investment by calculating its
Return on Capital Employed (ROCE) which is a ratio that represents how efficiently
a company turns a profit on its invested capital.
This project focuses on increasing the fidelity of the ROCE calculation using simulation
to project a range of possibilities for a given piece of equipment through its useful
life. Our team has developed an Excel-based decision support tool that allows an analyst
to compare various investment scenarios before proceeding in making an equipment purchase.
Statistical distributions are used to model key inputs in the ROCE calculation that
enables our tool to project confidence intervals representing possible financial return
from the investment over time. The decision support tool gives analysts a better understanding
for potential behavior of their equipment purchases before moving forward with a large-scale
capital investment.
Increase Profitability by Predicting Customer Operating Ratios using Machine Learning
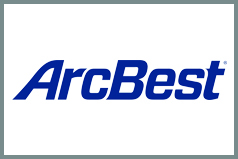
Team Members: Morgan Hartsell, Project Manager; Nikki Hayes, Jack Porter, Tad Dixon, and Parker King
Industry Partner: ArcBest
Industry Partner Contacts: Trenton Cason - Yield Analyst and Bradley Owens - Sr. Manager, Pricing and Supply Chain Analytics
ArcBest customers are offered an initial price from the pricing analyst department based on the provided information about the customers’ freight. The analysts determine the initial price, and potential profitability of a customer, through their expertise and evaluation of past accounts. This project involved data analysis of past shipments in order to provide a data-based tool for pricing analysts to use as a pricing aid. The Fully Allocated Operating Ratio (FAOR), represented by cost divided by revenue, was the key metric used to determine customer profitability. After baseline analysis, two machine learning algorithms, in the form of Random Forests, were implemented. The first iteration of Random Forests provided importance weights for the freight factors and how they influence FAOR. Another iteration of Random Forests was then used to calculate FAOR values and create a model based on past data, using the highest weighted factors as the input variables. The pricing analysts will be able to use the model by inputting specific freight factor values to then get a “Predicted FAOR”. The new systematic method and data-based approach will aid them in determining initial prices, leading to an increase in profitability for ArcBest.
Improving Outpatient Flow and Experiences by Implementing Simulation Techniques to Improve Resource Utilization
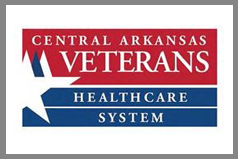
Team Members: Kevin Diaz, Project Manager; Andrew Clark, Lauren Curran, and Joy Jenkins
Industry Partner: Central Arkansas Veteran’s Health Care System
Industry Partner contacts: Amanda Furr - Chief Engineer, Amy D. Brown - System Redesign Coordinator; Precious Rife - GI Nurse Manager; Stephen Pettit - Program Manager and Jerry D. Meharg - MNSc, RN
Current endoscopy flow at the Central Arkansas Veterans Healthcare System (CAVHS) is based on outpatient surgical care approaches developed over forty years ago. Patients currently spend large amounts of time in a variety of time-consuming activities and locations, including check-in, holding, and recovery. The goal of this project is to generate a best practice model for patient flow, nurse and physician utilization, and space management for the CAVHS endoscopy unit. During this project, students created a time study sheet to gather sufficient data over the current process in the endoscopy unit. The students then used this data to analyze and create a simulation to give recommendations to the CAVHS endoscopy unit team with the goal of minimizing wait times, improving flow, and decreasing resource utilization for outpatient endoscopy. If successful, the project will save resources and money, decrease patient wait times, and reduce personnel deployment in the CAVHS endoscopy unit.
Reducing Internal Fuel Cost by Improving Operational Procedures
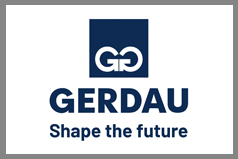
Team Members: Christian Faubel, Project Manager; Carter McDonald, Jasmine Richard, Steven Litwiller, and Alicia McComb
Industry Partner: Gerdau
Industry Partner Contacts: Clinton Johnson – Management Systems Facilitator, Shawn Hickman – Logistics Improvement Facilitator and Jason Mendez – Internal Logistics Routine Facilitator
The Internal Logistics Team at Gerdau’s Forth Smith mill facilitates the movement
of steel bundles throughout the Fort Smith facility from when they are first cast
until they are ready for customer delivery.
This project has been approached as a facility layout problem because the stockyards
are in essence acting as outdoor warehouses since they hold the bundles as inventory
between movements. This allows the yards to be defined in terms of rectilinear distances
and, therefore, mathematically analyzed. The best stockyard layout that was developed
sets criteria on the placement of bundles based on a bundle’s due date, which helps
standardize the movement of forklifts within the stockyards.
The first deliverable the Internal Logistics Team will receive is a new procedure
which serves as an operational instruction for material handlers to follow within
the stockyards. This allows the Internal Logistics Team to implement the new layout
over time without having to reorganize all the bundles that are currently located
in the stockyards. The second deliverable will be a decision support tool in Excel
that will update the team on the progress of correctly placed bundles as incorrectly
placed bundles leave the facility.
Streamlining the Parcel Rating Process using Customer Data Automation
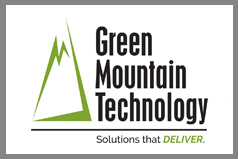
Team Members: Alec Hewitt, Project Manager; Vincent Chang, Ruby George, Mikki Harrell, and Blake Yates
Industry Partner: Green Mountain Technology
Industry Partner Contacts: Travis Peter - Strategic Solutions Engineer, Daniel Britton - Strategic Solutions Engineer and Katie Parker - Director of Strategic Solutions
This project serves to provide Green Mountain Technology’s Strategic Solutions Department with the ability to quickly and accurately estimate the parcel shipping costs across millions of shipments. Green Mountain Technology is a company that provides parcel data analysis through auditing and consulting to large ecommerce retailers. They work with both their clients and transportation providers to ensure that billing is consistent with contractual obligations. Green Mountain Technology also provides consultation using data analysis to locate areas where their clients can reduce their parcel spend. This project focuses on the consultation aspect of their business with the goal of reducing the amount of time required for Solutions Engineers to make a recommendation. To accomplish this, the capstone team utilized both Industrial and Computer Engineering concepts to create a decision support tool capable of automatically calculating shipping costs from customer data files. The processing of these files is complicated due to the complexities of each customer’s data files, resulting in Green Mountain Technology’s Solutions Engineers having to process files individually with a time-intensive data investigation process. With this solution and a supporting optimization model, the engineers will be able to quickly and consistently generate recommendations.
Supporting Product Innovation by Categorizing Products, Analyzing Flavor Profiles, and Defining Historical Trends.
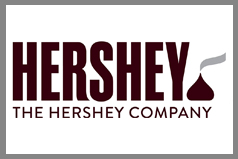
Team Members: Nicole Frear, Project Manager; Jalal Almuhanna, Abdul Almusaiteer, Ashley Johnson, and Kenny Phipps
Industry Partner: The Hershey Company
Industry Partner Contacts: Raegon Barnes - Director, Sales Strategy, Planning and Analytics, Walmart/Sam’s Club Sales, Robert Mancini - Sales Strategist – Sam’s Club and Willie Nelson - Sales Consultant - Walmart Seasons
The United States’ confectionery market is expected to grow at a rate of 1.68% annually
and earn $52.6 billion by 2025, according to Grand View Research Inc. The growth in
consumer demand and increasing competition requires that key vendors, such as Hershey,
Mars, and Nestlé, regularly introduce new flavors and ingredients into products. However,
one of the challenges associated with expanding product variety is to anticipate consumer
demand. Many companies collect vast amounts of information but lack a comprehensive,
user-friendly method to analyze it. Therefore, partnering with The Hershey Company,
this project uses Industrial Engineering techniques combined with data mining and
visualizations to establish a foundation for consumer trend analysis.
The Strategy, Planning, and Analytics (SPA) team at Hershey’s Bentonville office performs
data analysis to understand product performance and cyclical trends. However, the
team struggles with inconsistent product groupings and flavor descriptions. Thus,
this project seeks to accurately and completely re-categorize products by flavor.
K-means clustering for machine learning forms hierarchical links between products
containing similar attributes. An optimization model groups the product clusters into
categories that most specifically identify the flavor composition. Descriptive statistics
are displayed on an interactive Tableau dashboard so Hershey gains insights and makes
informed decisions for the future.
Identifying Post Seasonal Missed Sales Opportunities using Automated Data Analysis
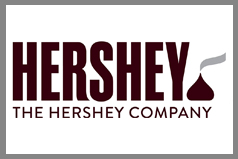
Team Members: Bria Garcia, Project Manager; Brooke Larkin, Ty Martindale, Hector Ramos, and Alejandro Torrico
Industry Partner: The Hershey Company
Industry Partner Contacts: Raegon Barnes - Director of Sales Analytics for Walmart/Club Team and Willie Nelson - Sales Consultant for Walmart Seasons
The Hershey Company is a global snacking powerhouse and the undisputed US leader in confection, producing iconic products like Reese’s, Hershey’s Kisses, and Jolly Ranchers. Not only does The Hershey Company produce these products year-round, but they also have a large portfolio (market share leading) in four traditional seasons: Valentines, Easter, Halloween, and Holiday. The Strategy, Planning, and Analytics (SPA) team at the Bentonville office is tasked with identifying missed sales opportunities (MSOs) after each completed season. The process of identifying MSOs is manual, subjective, and takes a significant amount of time. To address these issues, our team created a web-based application using the scripting language, R. This tool automates the process of identifying MSOs using three methodologies and displays the results in a variety of visuals via the user interface. As a result, the tool significantly decreases the time of the analysis. To further aid the SPA team, our team created an optimization model that outputs the optimal quantity of each product. The Hershey Company should have sold to Walmart for each season to maximize Walmart profit. This is an extra selling point for The Hershey Company since it gives them insight into which products benefit Walmart the most.
Improving Load Planning by Modeling Variability in Driver Activity Time
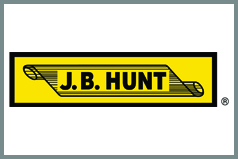
Team Members: Emily Matlock, Project Manager; Majed Alahmadi, McKenzie Barnes, Hunter Chastain, and Sam Jeffcoat
Industry Partner: J.B. Hunt Transport, Inc. (Intermodal Division)
Industry Partner Contacts: Jordan Sonnentag - Logistics Engineer and Alex Wong - Logistics Engineer
Load planning is a crucial task within J.B. Hunt Intermodal’s operations. Without efficient and reliable load planning, J.B. Hunt suffers the risk of late or missed deliveries, which would negatively impact their levels of service and their operational efficiency. Load planning is the process in which Logistics Coordinators assign customer-ordered loads to drivers to be transported. Logistics Coordinators use the following time estimates when assigning loads to drivers: transit time, scheduled load appointment time, driver estimated time of availability, and driver activity time (DAT). Our project seeks to provide a better method of estimating DAT to improve the load planning process overall, leading to more accurate scheduling for follow-up loads, increased service, and lower operational costs. DAT is the time a driver spends at a customer location either picking up or dropping off a load. The current method of estimating DAT is to take the average DAT per customer over a specified period of time, usually 6 weeks. This method fails to account for the inherent variability of DAT, so our team has formulated a predictive model that uses several predictor variables to provide a better DAT estimate.
Improving the New Business Opportunity Process Using a Decision Support Tool and Optimization Model
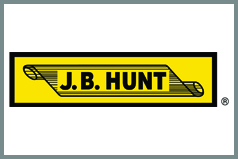
Team Members: Kaitlin Smithey, Project Manager; Coleman Crouch, Jaylan Matthews, Tara Minmier, and Reid Skinner
Industry Partner: J.B. Hunt Transport, Inc.
Industry Partner Contacts: Christopher Schock - Senior Logistics Engineer, Alex Andelman - Regional Engineer and Tony Woods - Associate Logistics Engineer
J.B. Hunt Transport Inc. is a Fortune 500 company in the transportation logistics industry offering services to a diverse set of customers. The Dedicated Contract Services (DCS) division of J.B. Hunt was created to provide outsourcing solutions for customers, customizing fleet operations to the customer’s needs. When developing a proposal for a new customer, Design Engineers create a recommendation for equipment and drivers. Historically, this process relied on the Design Engineer’s intuition and each account was treated separately which resulted in resource-heavy recommendations. We created a decision support tool combined with a linear optimization model to improve this process and reduce the cost of recommendations. Our decision support tool utilizes VBA code and R Studio to perform analysis on driver skill level, seasonality, and equipment similarities between DCS customer accounts. The optimization model considers these same components to cluster similar accounts to validate the performance of the tool.
Improving Forecasting Accuracy of Intermodal Pickup Demand by Integrating Statistical Forecasting Methods
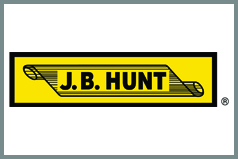
Team Members: Luke Turner, Project Manager; Dustin Adcock, Payton Moore, Olivia Ohlstein, and James Waller
Industry Partner: J.B. Hunt Transport, Inc.
Industry Partner Contacts: Alex Wong - Logistics Engineer and Jordan Sonnentag - Logistics Engineer
J. B. Hunt Transport, Inc. Intermodal (JBI) division holds the highest percentage of the company’s revenue and has experienced considerable growth in the last 10 years, their orders nearly doubling. Significant planning and preparation are involved in allocating Intermodal containers across the rail network. To handle this growth along with network uncertainty, a predictive approach is not only desirable, but also necessary. Currently, JBI’s network planning team projects the demand of containers by operating regions using intuition. In an effort to develop a standardized and statistical approach to forecast their demand, we created an optimization model to interpolate five different forecasting methods from Holt-Winters, Auto-Regressive Moving Average, Weekday Running Average, Lead Time Orders, and Socioeconomic Regression. We integrated our method into an R-Script and created a dashboard proof of concept for the network planning team to employ. When compared to their original approach, our optimization model produces a more accurate forecast, in a fraction of the time. In future, JBI will implement our R-script into their systems to aid in their forecasting process.
Automating Product Selection and Removal Process for Cross Dock Operation via Decision Support Tool
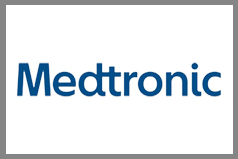
Team Members: Nate Hemby, Project Manager; Kyle Adams, Tylon Jackson, Damon Pettis, and Ben Thornton
Industry Partner: Medtronic PLC
Industry Partner Contacts: Guido Malpartida - Supervisor/Lead Program, Global Planning and Mike McCamish - Senior Manager, Global Planning
Medtronic is a global medical device manufacturing company with major supply chain operations to support the manufacturing effort. Our project is a part of a larger project headed by CELDi that is focused on supporting the Medtronic cross dock in Pedrickstown, NJ. The overarching CELDi project is examining the current parameters by which Medtronic selects products to flow through the cross dock and trying to determine the most cost-effective parameters for selecting products for the cross dock. For our part of the project, we were tasked with designing a product selection tool to help streamline the selection process. Much of the current process is done manually, with large portions of the process being spreadsheet manipulation. We sought to build a tool that would seamlessly integrate into the current process, automating large portions of the selection process. Using the tool is expected to yield a 50-60% reduction in the process time, saving Medtronic labor costs and freeing up workers to focus on more value-added activities within the company.
Improving Staffing and Resource Allocation through Forecasting of Emergency Department Patient Volumes and Admission Rates
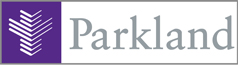
Team Members: Matt Millis, Project Manager; Jake Harrison, Parker Galligan, Josh Jensen, and Cory Stewart
Industry Partner: Parkland Health & Hospital System
Industry Partner Contacts: Nina Drolc - Operational Excellence Project Manager Analyst, William Murray - Dir. of Operational Excellence Project Management and Gabriel Fruge - Project Manager, Transformational Initiatives
Parkland Health & Hospital System’s Emergency Department is one of the busiest in the nation with around 250,000 patients visiting annually. Emergency Department patient volumes can swing substantially (±40%) which can affect day-to-day operations. These volumes impact Parkland’s Bed Access Management Team, who handle the allocation of beds to admitted patients, and Nursing Services, who deal with staffing throughout the hospital. Our team sought to provide Parkland with the ability to make informed decisions related to hospital-wide staffing and resource allocation by forecasting patient arrivals and admissions through the Emergency Department using predictive analytics. We developed a multiple linear regression model with predictors representing Day of Week, Month, and Holidays. We were able to forecast patient arrivals and admissions as far as one year in advance. We have delivered our one-click update forecasts to Parkland including the evaluative metrics Mean Absolute Percent Error and Extreme Error Days. We anticipate these forecasts to have an impact on patient wait times and service levels throughout the hospital.
Increasing Plant Productivity by Improving the Current Scheduling Process
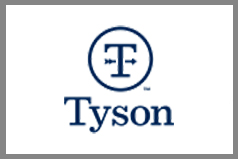
Team Members: Dasher Smith, Project Manager; Andrew Burroughs, Elisa Daniel, Oscar Guzman, and Joe Thongsovath
Industry Partner: Tyson Foods
Industry Partner Contacts: Marc Schwartz -Associate Director of Continuous Improvement and Craig Short- Director of Continuous Improvement
The purpose of our project is to help Tyson Foods improve production opportunities in their Shelbyville, Tennessee chicken tray packing plant. The current manual scheduling process yields a higher number of changeovers and experiences an imbalance of production across all packaging lines leading to increased idle time and non-value-added activities. Our team explored two alternatives to approaching the problem. The first approach utilizes a global optimization approach in the form of an integer program to allocate and sequence orders to packaging lines with an objective of minimizing the total changeover time. However, due to the anatomy of the chicken and the complex production process, we found that the problem lent itself to be more suitable for our second alternative: a decision support tool, which allowed for the level of detail required to seek a feasible solution. Our decision support tool takes a sequential optimization approach in which we allocated orders using a variation of a bin packing heuristic and then sequence the orders by grouping like tray sizes together to reduce changeover time. The tool creates a schedule and outputs three key performance measures: total changeover time, total changeover cost, and machine utilization used to assess productivity and efficiency improvement.
2nd Annual Industrial Engineering Capstone Symposium
Increasing Business Won and Reducing Turnaround Time by Modifying ArcBest’s Bid and Proposal Processes
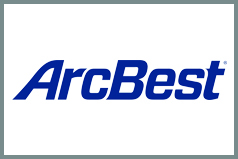
Team Members: Trenton Cason, Project Manager; Monica Briselden, Adrew Doner and Brittany Miller.
Industry Partner: ArcBest Corporation
Industry Partner Contacts: Philip Marsh, Senior Yield Analyst and Brad Owens, Pricing Manager
Less than truckload (LTL) transportation is an industry focusing on delivering loads that do not require a full 48 or 52 foot trailer, but rather a 28 foot trailer that can be filled with multiple orders to be dropped off along a driver’s route. ABF Freight, ArcBest’s LTL subsidiary, is seeking to improve their business processes to analyze new business decisions. Today, ABF Freight (ABF), a premium carrier in the industry, only wins a relatively small proportion of these opportunities. This could be due to poor inter-team communication, a lack of process performance visibility, and a lack of prioritization of the deals that they receive daily as well as their position as a premium carrier in the market. After detailed analysis, it was determined that a glossary and new justification form could be used for improving communication and a logistic regression model to prioritize each new deal to save time and reduce turnaround time.
Calculating Forklift Replacement and Assessing Alternatives
Team Members: Brian Meyer, Project Manager; William Beckman, Nicolas Rork and Tyler Wiggins.
Industry Partner: ArcBest Freight
Industry Contacts: Kriby Clark, Sr. Manager Financial Analysis, Engineering & Pricing
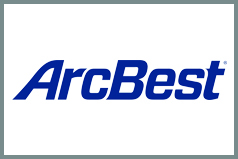
ABF Freight’s forklift fleet is critical to their day – to – day operations. Forklifts allow dock workers to quickly unload and load trailers at their distribution centers and end – of – line facilities. These are used throughout the day, 7 days a week, 52 weeks a year. Thus, most forklifts will accumulate a high number of engine hours throughout its life. ABF Freight’s current policy is to retire forklifts after 8,000 to 10,000 engine hours. The retirement of forklifts, and subsequent purchase of new forklifts, needs to be financially justified. To access whether a forklift should be retired, the project details the calculation of multiple optimal replacement intervals, as well as the creation of a tool that recommends if a forklift should be retired or not by clustering forklifts based on their operation and maintenance cost. ABF Freight also wants to know if there is a different forklift type better suited for their operations. Currently, ABF Freight uses propane powered sit – down counterbalance forklifts and is considering switching to electric. The project also details a multiple objective decision analysis which recommends the best forklift alternative based on value measures provided by ABF Freight.
Increasing Cross-Dock Efficiency by Improving Door Assignments
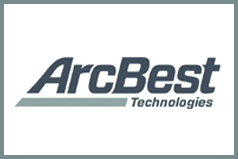
Team Members: Aaron Bowden, Project Manager; Charles Elliott, Royal Hart and Olivia Weekley.
Industry Partner: ArcBest Technologies
Industry Contacts: Brett Spicer, Manager, Advanced Analytics and Chris Crisanti, Lead Engineer
ArcBest Technologies serves as a subsidiary to ArcBest Corporation and their main focus is to support the technology initiatives of all ArcBest business units, including ArcBest subsidiary ABF Freight. ABF Freight has multiple cross-dock service centers across the country where countless products are received and shipped every day. A key aspect of improving the efficiency of these cross-docks is to properly assign outbound and inbound locations to certain doors in the service center. Traditionally, the service center managers use their experiences and prior knowledge to make these decisions, but ArcBest Technologies has decided they want a tool to verify if these changes will be successful prior to execution. The best way to validate success is to build a simulation model that can accurately represent the day-to-day operations of any service center and can be adjusted to fit any layout of the door assignments. This will allow the managers to make the changes, run them through the simulation model, and analyze the output metrics such as distance traveled, bills per dock hour, and facility congestion. Because of its adaptability, ArcBest Technologies should be able to benefit from this simulation model for many years to come.
Improved Food Production Operations: Advancements in Facility Layout, Job Scheduling and Data Management
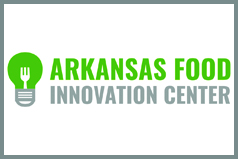
Team Members: Justin Taylor, Project Manager; Brisa Bartczak, Karla Castro and Craig Gifford.
Industry Partner: Arkansas Food Innovation Center
Industry Contacts: John Swenson, AFIC Manager
The Arkansas Food Innovation Center (AFIC) is a facility operated through the University of Arkansas Division of Agriculture Research & Extension that aids local entrepreneurs in commercially producing their food products. Within the facility, operations are run almost entirely by the manager, Mr. John Swenson. In an effort to reduce the amount of non-value added activities performed by Mr. Swenson on a daily basis, this project focuses on three objectives relevant to the operations of the facility: layout, scheduling, and data storage. While taking constraints such as machine movability into account, a new layout was developed to increase the distance-based efficiency of the facility. A decision support tool was created to automate the process of scheduling producers based on time and machine availability. Lastly, a data storage solution was designed to organize facility information, provide relevant information for the scheduling tool, and serve as a repository for future AFIC facilities. Accomplishing these three objectives not only improves the functionality and efficiency of the facility, but allows for the reallocation of Mr. Swenson’s time towards helping the food entrepreneurs of Northwest Arkansas.
Improving Accuracy of Appointment Capacity Forecasting Utilizing Predictive Modeling
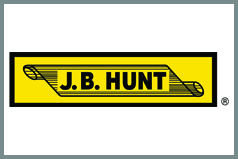
Team Members: Brittany Watts, Project Manager; Amada Beard, Seth Briley and Matthew Turner.
Industry Partner: J.B. Hunt
Industry Contacts: Brent Wiles, Logistics Engineer and Emily May, Associate Logistics Engineer
J.B. Hunt Transport’s Intermodal division (JBI) services customers all throughout North America, utilizing strategic partnerships with 5 primary rail companies, in conjunction with their trucks. JBI has grouped their customers into over 130 markets. Many of these markets rely on an appointment capacity forecast to manage freight deliveries daily. Our team is focused on improving the forecasting model to more accurately estimate how many delivery appointments can be completed in a market. JBI’s current model creates estimates using historically voluminous days to act as a capacity limit, and focusing on historic availability causes the estimate to be a lagging metric. Our team created a mathematical model focused on the limiting resource of driver time to predict appointment capacity. Our model also includes a procedure for incorporating a single appointment’s impact on the market’s total appointment capacity. Our team’s model produces an estimate that incorporates changing market characteristics with historical data through focusing on the number of drivers available in a market and considering the fact that not all appointments require the same amount of time.
Increasing Tractor Utilization Using Scripted Integer and Linear Programming
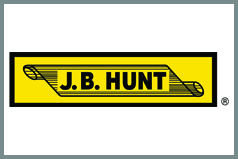
Team Members: Grace McGee, Project Manager; Danarrius Broadway, Anginay Jones and Garrett Tallman.
Industry Partner: J.B. Hunt
Industry Contacts: Adam Klausing, JBI Logistics Engineer and Alex Wong, JBI Logistics Engineer
J.B. Hunt is a transportation logistics company that offers services to a large range of customers across North America. The Intermodal business unit of J.B. Hunt (JBI) partners with six major rail carriers to provide intermodal freight solutions to customers. Their associated tractor fleet is majority company owned, giving JBI control over the drayage segment of an intermodal load. To accommodate volume growth, JBI traditionally increases their tractor fleet annually, relying on volume forecasts and subject matter expertise to justify tractor need. JBI recognizes the opportunity to improve this decision-making process by modeling their current fleet utilization and baselining the potential fleet utilization per location. To address these needs, our team created an optimization tool using the scripting language, R. This tool minimizes the variance of appointments completed per hour by redistributing historic customer appointments incrementally using integer programming. The tool then uses linear programming and the newly distributed data to develop a driver schedule capable of completing all customer appointments on time. The resulting metrics are used to provide a proper baseline for a location’s tractor utilization.
Predicting the Cost of Driver Pay to Increase the Accuracy of Future Bids
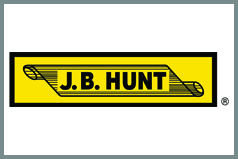
Team Members: Caine McLeod, Project Manager; Jackson Goodman, Zachary Osiecki and Foster Pollock.
Industry Partner: J.B. Hunt
Industry Contacts: Joey Castrodale, Sr. Associate Logistics Engineer and Marc Alley, Sr. Director Engineering and Technology
The trucking industry, as a whole, has a very high turnover rate for drivers. The turnover rate can vary wildly from 40% to as high as 200% on an annualized basis. When bidding a contract for potential customers, estimating the cost of driver pay can be very difficult because of the volatility in the market. Currently, J.B. Hunt relies heavily on industry experts to estimate these costs. Our team decided to create a decision support tool to help ground these estimates in two sets of data. The first set, J.B. Hunt’s internal data, was analyzed using many different data analysis techniques to determine an appropriate regression model for predicting driver pay. The second set, from the Bureau of Labor Statistics, is automatically collected and used to validate each prediction. The end-user is presented with a summary of the analyses performed, so he or she is better equipped to make an estimate.
Instituting a Worker Scheduling Tool for the Pre-molding Process
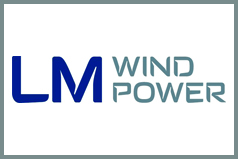
Team Members: Anna Hudgeons, Project Manager; Shay Brown, Austin Kuklenski and Cody Lawrence.
Industry Partner: LM Wind Power
Industry Contacts: Erin Lanford, Manager, Cycle Time Projects Global Production Engineering & Implementation
LM Wind Power, a GE renewable energy business focused on the manufacturing of wind turbine blades, has introduced a cycle time reduction strategy known as “pre-molding”. In this system, materials are constructed ahead of schedule to make their implementation into the mold as efficient as possible. This project focuses on the creation of a worker scheduling tool that aids in determining the allocation of resources for LM’s pre-molding operations based on weekly blade demand. Components of the project include time studies, data analysis, optimization, knapsack scheduling, and VBA coding.
Increase Productivity in the Molding Process Through a Blade Tracking Tool and Identification of Main Causes for Rework
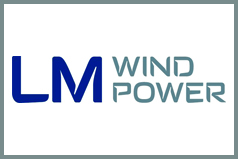
Team Members: Maria Camila Ruiz, Project Manager; Jose Bracamonte, Daniel Ostrand and Barbara Stanziola.
Industry Partner: LM Wind Power
Industry Contacts: Erin Lanford, Manager, Cycle Time Projects Global Production Engineering & Implementation
LM Wind Power is the leader in the blade manufacturing industry for wind turbines around the world. The team is working with their Little Rock facility in order to look for opportunities where they can increase their blade output in the molding process and decrease lost productive time. LM wanted a tool that can give an overview of the current status of the process, that is adaptable to be used in other facilities, and that can help supervisors with quick decision-making. As a result, a dashboard that is both interactive and visually appealing was created using Microsoft Excel and Visual Basics and it complies with the key characteristics LM required. Extensive data analysis was also performed to identify the main defects that cause over-time in the closing phase. Historical data was analyzed using Minitab to identify the correlation between the number of defects and the closing times. Based on this information, LM can focus on reducing specific defects to reduce the overall manufacturing time.
Providing Insight on Automated Passenger Counting Data to Improve Bus Utilization
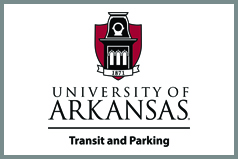
Team Members: Allison Morast, Project Manager; Blake Dougan, Victoria Goethel and Austin Talley.
Industry Partner: University of Arkansas Transit and Parking
Industry Contacts: Gary Smith, Director, Transit and Parking, Adam Waddell, Associate Director for Transit and Colton Duke, Logistics Manager
Razorback Transit has subscribed to automated passenger counting (APC) systems, which count the number of passengers boarding and alighting (getting on and off) a bus at each bus stop. The APC systems are installed on each of Transit’s 25 buses which operate on 16 different routes that travel throughout Fayetteville. Each time a bus operates, data collected from the APC systems is sent to a website where it can be downloaded and then analyzed. This project presents a method for analyzing this data to evaluate and change current bus routes to help increase bus utilization. This is increasingly important because the University of Arkansas has experienced enrollment growth every year for more than two decades with no foreseeable recession. As a result, parking demand is expected to outgrow supply, meaning that Razorback Transit will need to improve bus utilization in order to serve the growing number of students. To help Razorback Transit accomplish this, we have created an interactive Tableau dashboard that calculates key performance indicators and visual aids, as well as a predictive model of passenger demand that will assist Razorback Transit in deciding where to place new bus stops.
Simplifying Campus Parking for Increased User Satisfaction and Improved Decision Making
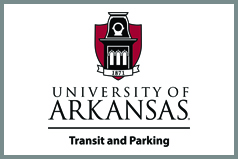
Team Members: Ryan Sanders, Project Manager; Mireille Ineza, Christopher Manjarrez and Jacob Washkowiak.
Industry Partner: University of Arkansas Transit and Parking
Industry Contacts: Gary Smith, Director, Transit and Parking, Debbie Woods, Business Manager and David Wilson, Communications Director
The goal of this project is to work with University of Arkansas Transit and Parking in order to simplify campus parking for increased user satisfaction and improved decision making. Stakeholder analysis revealed high levels of dissatisfaction towards the current parking system and a lack of efficient communication of complaints within the Transit and Parking Department. Extensive data analysis showed the need for drastically reducing the complexity of the parking system. The objectives of this project were to (1) increase satisfaction of people who park on campus, (2) reduce the complexity of the University of Arkansas’s current parking system, and (3) improve visibility and communication. These objectives were accomplished through (1) performing decision analysis to determine the best method of permit holder communication, (2) using cost analysis and a calculated parking lot complexity score to make recommendations for beneficial changes to the parking system, and (3) implementing a database to track and report on complaints received.
Improving Patient Flow by Reducing Emergency Department Length of Stay
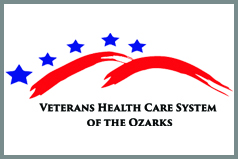
Team Members: Olivia Goss, Project Manager; Brooks Langdon, Ali Madere and Domenic Pacitti.
Industry Partner: Veteran’s Health Care System of the Ozarks
Industry Contacts: Amy Lesniewski, RN, MS, Assoc. Director Patient Services, Michael T. Anderson, MHA, Health System Specialist, David Cavins, RN, CCEMT-P, Emergency Dept. Nurse and Tiffany Goodwin, Executive Assistant
The Veterans Health Care System of the Ozarks (VHSO) provides high quality health care services to 55,000 plus Veterans living in and visiting 23 counties in northwest Arkansas, southwest Missouri, and eastern Oklahoma. Currently, their Emergency Department is experiencing a patient length of stay of around 200 minutes. Our project team has been tasked to increase access, reliability, and service quality within the Emergency Department. To assess the performance of the solutions in accordance with our issue at hand, the patient length of stay will be the value metric used to evaluate the success of each modification. For this work, five deliverables have been established: work sampling, data analysis, methods and standards, facility layout, and portfolio analysis. Combining our team members Industrial Engineering skills, the VHSO staffs’ knowledge, tools such as simulation software, and data provided, these deliverables will create an outline of modifications with cost/benefit ratios that the VHSO can make in their Emergency Department to reduce their patients’ length of stay.
Reducing Cardboard Contamination Throughout the VHSO by Redesigning the Facility Layout
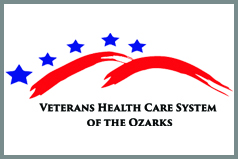
Team Members: Fallon Haaser, Project Manager; Aliyah Conley, Brian Sloan and Deondre Taylor.
Industry Partner: Veteran’s Health Care System of the Ozarks
Industry Contacts: Michael Anderson, Health Systems Specialist and Andrew Deluise, Chief of Inventory Management
The Veterans Health Care Administration is the largest integrated healthcare system in the United States with over 1,243 healthcare facilities around the nation. The Veterans Health Care System of the Ozarks (VHSO) is the local VA hospital in Fayetteville, AR and is rated as a tier one facility due to their advanced mental health facilities. There have been recent studies to show that cardboard is a contaminant in hospital sterile areas. The VHSO’s current receiving and breakdown process has cardboard cycling near sterile and non-sterile inventory storage areas, where cardboard is prohibited. The VHSO has tasked us with redesigning the facility layout of their current supply room, while being in compliance with VHA Directives 1116 and 1761. Industrial engineering skills were used to determine and analyze two viable alternative processes for completing the redesign of the receiving and breakdown process, providing an economic justification for both.
Tracking Goods Not for Resale in the Backroom of Walmart Stores
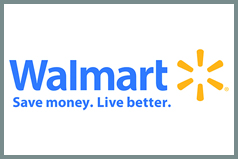
Team Members: Peter Scheele, Project Manager; Nour Abu-Safe, Savanna Kis and Timothy O'Toole.
Industry Partner: Walmart Inc.
Industry Contacts: Matthew W. Foster, Director, Procurement Services and Spend Analytics and Anshuman Awad, Senior Manager I, Global Business
Our team has analyzed demand data for goods not for resale (GNFR) and researched more efficient ways to measure consumption in the backroom of Walmart stores. GNFR includes plastic bags, toilet paper, paper towels, cleaning supplies, and other supplies purchased by a store that are not sold to consumers. Tracking GNFR items is often overlooked by retailers due to their low frequency and relatively low price. However, inefficient management of these goods leads to excess spending in inventory holding and ordering costs. Some technical alternatives that we have researched include implementing RFID technology and video recognition/analytics. In addition to evaluating each of these potential systems, we developed a lot size, reorder point system for a subset of their most ordered items. The transition from a periodic inventory model to a perpetual inventory model will allow Walmart to reduce labor time while increasing their inventory accuracy. Our objective is to evaluate the cost versus value of these different system alternatives so Walmart can obtain accurate inventory levels.
Revamping Workforce Management Processes for Increased Associate Utilization
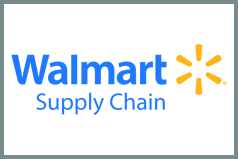
Team Members: Anthony Woods, Project Manager; Spencer Kilgore, Hunter Pauling and Grant Waller.
Industry Partner: Walmart Supply Chain
Industry Contacts: Jaclyn Johnson, Project Manager, Supply Chain Engineering and Kyle Kimpel, Senir Director, Supply Chain Engineering
Walmart Inc. is a Fortune 1 company with yearly revenues of over $480 billion and over 11,500 stores across 28 different countries. To keep general merchandise products replenished in stores, products must efficiently and effectively flow through 42 regional distribution centers (RDCs) across the United States. Because Walmart’s associates are the most crucial piece to its successful operations in these regional distribution centers, Walmart is interested in continually improving their workforce management processes in their RDCs. This project focuses on analyzing the areas of staffing decisions, retention, productivity, and process simplification to improve operations and reduce costs in Walmart’s RDC in Bentonville, AR, DC 6094. First, simulation modeling was used to help managers make better staffing decisions, ensuring the distribution assembly receiving department is efficiently staffed. Next, data analysis methods were used to gain insights into how to decrease associate resignations and increase associate productivity levels. Finally, a data transfer process for managers in distribution assembly receiving was redesigned and improved, saving roughly 1.5 hours of time every day and improving data accuracy for Walmart. Overall, this project and analysis will help Walmart reduce costs, better support their associates, and serve their customers better.
Improved Decision Support in Walmart’s Fresh Produce Modular Design
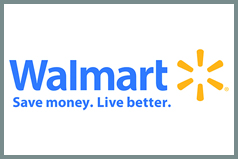
Team Members: Chase Kilty, Project Manager; Cherzulyn Garcia and Elizabeth Laster
Industry Partner: Walmart, Inc.
Industry Contacts: Nicole Ryner, Director, Modular & Space Data Strategy, Keith Tisdale, Senior Manager II, Analtytics Category Space Modular Development Produce
Walmart entered the grocery business in 1988 with the desire to become a one stop shop for customers worldwide. Grocery has grown into Walmart’s largest merchandise unit and is responsible for over half the company’s annual revenue. Within grocery, Walmart’s fresh produce is responsible for approximately $12.5 billion dollars in annual sales and is essential for driving store traffic and repeat visits from customers. However, produce creates perishable inventories that drive waste and Walmart designs their fresh produce sections to reduce this waste while also balancing the occurrence of stock outs. Our team has developed a decision support tool that will aid Walmart in their modular design process by identifying produce stock keeping units (SKUs) that are underperforming. The tool takes historical sales data from store clusters and calculates weekly averages of sales, dollars lost due to waste, and throw percentage per SKU. It then indexes these values against the Walmart average to identify SKUs that need further attention from modular design managers. The tool’s output enables better informed decision making when determining the amount of space to allocate to each SKU within Walmart’s fresh produce departments.
Reducing Manual Decisions in the Trailer-to-Door Allocation Process
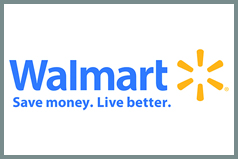
Team Members: Teresa Nguyen, Project Manager; Carson Alsup, Sarah Belcher and Ricky Ng
Industry Partner: Walmart, Inc.
Industry Contacts: Annie Fuentes, Sr. Dir. Supply Chain Central Operations, Mike McCage, Sr. Manager, Supply Chain Product & Tech. and Adam Cline, Product Manager, Supply Chain Product & Tech.
Working with Walmart’s Supply Chain Team, we are focused on increasing efficiency by reducing manual decisions made by managers in the receiving area of the Walmart’s distribution centers. Currently, managers must manually allocate inbound trailers to an available dock door. Attempts to automate the process have been poorly received. Our project involves modeling the decision process and providing support to receiving managers by combining the logic of seasoned Walmart associates and industrial engineering methodology. Developed in Excel VBA, the support tool uses information from Walmart’s Yard Management System to get real-time data on inbound trailers. Door recommendations are given based on defined logic and constraints. For the staple stock dock, the objective is to minimize the haul distance between where a trailer is unloaded and the freight’s storage location in the warehouse. For the distribution assembly dock, where there are three mechanisms for receiving freight, the objective is to place a trailer where it can be best serviced by the most appropriate mechanism based on the type of freight. The support tool helps receiving managers make better, faster, and fewer decisions. It reduces the necessity of experience, greatly improving the performance of new managers, and productivity of the warehouse will improve by reducing hauling distances.
Redesigning Dry Grocery Category Adjacency
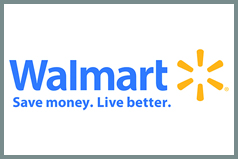
Team Members: Jennifer Watson, Project Manager; Kelly Green and Bryce Rohr
Industry Partner: Walmart, Inc.
Industry Contacts: Nicole Ryner, Director, Mod Dev Data Strategy and Matt Yarbrough, Sr. Manager, Category Space Support
The first Walmart Supercenter opened in 1988 combining a full grocery section with a general merchandise store. Within the Grocery department, our project focus is on the Dry Grocery section, which consists of pantry-type foods such as flour and canned goods. Since 1988, Dry Grocery has seen little to no standardization or changes regarding the product placement of categories of foods. Our Capstone team was tasked to understand the current performance of the Dry Grocery section and to develop a layout by analyzing transactional data. The goal was to mathematically arrange the products such that the customer will be satisfied and enjoy a more efficient shopping experience. Market basket analysis is a modeling technique that is used to understand the relationship between products that customers buy. This analysis technique, combined with the distance between categories was used to develop a new metric that represents the correlation between category pairings. Using our metrics and defined adjacency measures, we were able to develop a layout that increased customer efficiency. The team also developed a decision support tool for Walmart to use to aid in further layout decisions in Dry Grocery which is adaptable to other departments as well.
Allocating Products to Channels in the Item Discovery Funnel
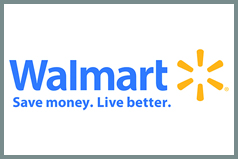
Team Members: Rachel Holmer, Project Manager; Harrison Gilker and Juan Marino
Industry Partner: Walmart, Inc.
The channel through which Walmart decides to provide products to consumers has a large impact on the success of the business. There are four channels available for Walmart to choose: marketplace, direct ship from vendor, owned, and store. Each channel possesses different levels of financial investment for Walmart. Marketplace has the least amount of financial investment, with Walmart solely receiving a commission based on every item sold through the channel. The store channel has the highest level of financial investment, with Walmart taking ownership of all costs from the distribution centers to final sale. To aid Walmart with proper allocation, we have modeled the decision-making process that current buyer’s go through to place the product into Walmart inventory. After consulting with our main stakeholders, we then developed a tool using Excel VBA to perform this decision-making process for buyers. This tool simplifies the approach that buyers take on allocating items while also eliminating the presence of bias from the buyer. After testing to ensure the tool logic is sound, our deliverable will be given to Walmart to implement into their business structure.
1st Annual Industrial Engineering Capstone Symposium
Creating Data Driven Guidelines to Improve ABF Freight’s Contract Negotiation Process
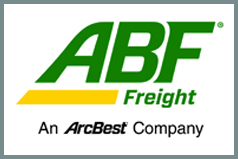
Team Members: Nina Drolc, Project Manager; Elizabeth Carter, Alex Huckabee and Tanner Woodruff
Industry Partner: ABF Freight
Industry Contacts: Christopher Adkins, Sr. Yield & Innovation Analyst and Bryan Johnson, Yield Manager
ABF Freight pricing analysts and salespeople must cooperate to negotiate thousands of pricing agreements with customers each year. While some customers agree on predetermined yearly price increases, others choose to defer to periodic negotiations. Deferred contracts require annual renegotiation called Pricing Proposal Maintenances, or PPMs. The pricing analyst assigned to a PPM will discuss pricing strategy with the salesperson, who ultimately works with the customer to reach an agreement. Currently, pricing strategy is predominately built on personal experience and contract history. However, PPMs can be uniquely described by a number of other characteristics such as customer relationship, account size, price sensitivity, market conditions, salesmanship, requested increase amount, etc. These characteristics were evaluated using regression for their ability to predict future outcomes. The change in price and retention of business, which indicate the success of a PPM, were used as response variables in the analysis. Linear regression was used to assess the effect of predictors on the size of the change in price. In addition, logistic regression was used to assess the plausibility of getting an increase and risk of not retaining the business. Conclusions from our analysis were used to design a decision support tool and establish data driven guidelines to improve the results of future PPMs and consequently increase ABF Freight’s profit.
Improving Labor Scheduling while Considering Cost
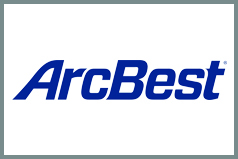
Team Members: Alex Laguarta, Project Manager; Stephanie Gibbs, Tyler Morris, and Reagan Villarreal
Industry Partner: ArcBest
Industry Contacts: Brett Spicer, Lead Business Insight Analyst and Robert Wittig, Director, Business Insight & Analytics
We have improved dock labor scheduling at the South Chicago terminal maximizing both employee efficiency and profitability. Improving the labor scheduling at the DC will not only cut operating expenses, but also help ABF better serve their current customers and potentially attract new customers. Labor scheduling is crucial to a distribution center because if there are too many employees on the dock, employee efficiency decreases, and the dock becomes oversaturated. Employee idleness may occur, and ABF incurs greater labor expense than expected. However, if there are not enough employees present, terminal bottlenecks, missed appointments, and upset customers may result. Currently, operation managers create a bid schedule every six months. Regular full-time employees select their shifts based on seniority. However, occasionally more help is needed on certain days. Therefore, operation managers must call in a casual employee. The bid schedule is made using gut feelings and intuition, which yields a high percentage of variability in the total number of employees on the dock versus the number of bid employees who are scheduled per day. We have developed a labor scheduling deliverable based on forecasted incoming and outgoing bills to maximize both employee efficiency and profitability. We created a decision support tool that improves the bid schedules based on forecasting. We used historical data regarding incoming and outgoing bills to forecast the demand at the terminal over a period of six months. An optimization model uses this forecasted demand as a parameter to determine an efficient and effective bid schedule.
Reducing Costs by Improving Efficiency in Product Sorting and Delivery Processes
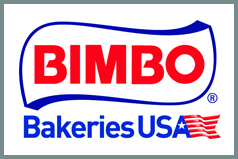
Team Members: David Cox, Project Manager; Levi Finn, Daniel Ramirez, and Cristhian Rojas Quintero.
Industry Partner: Bimbo Bakeries USA
Industry Contact: Roberto Arizmendi, Director of Engineering
Bimbo Bakeries USA (BBU) utilizes multiple facilities to carry out its logistics, which make up their overall supply chain. The last facility in this supply chain is known as a business center, and multiple bakeries feed these business centers. At business centers, products are unloaded from inbound trailers, sorted by delivery routes, further sorted by destinations, loaded onto delivery trucks, and delivered to BBU’s clients, such as Walmart stores, Sonic restaurants, schools, and family-owned restaurants. These operations are done manually, so the efficiency of its workers directly impacts the cost of running this facility. This project seeks to provide understanding of the business center’s system, discover its inefficiencies, provide analysis-based recommendations to alleviate the inefficiencies, and deliver continuous improvement tools to BBU. Multiple findings have arisen: the current unloading and sorting processes are not standardized; the layout of the business center’s sorting process experiences avoidable congestion; the process used to sort products by their destinations is not standardized; practices for collection data on delivery are not standardized; the necessary workload to deliver product at stores is not considered when routes are designed. These findings resulted in the creation of a simulation model, time study, workload model, and data collection guide to provide BBU with recommendations and tools for continuous improvement.
Maximizing FedEx Freight’s Dock Performance through Efficient Work Assignment Methods
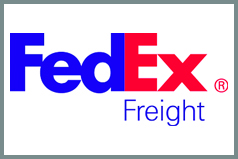
Team Members: Elizabeth Schad, Project Manager; Emily Daniel, Barth K. Onyekwelu, and Brody McDonald
Industry Partner: FedEx Freight
Industry Contact: Kiran Chittoori, Sr. Advisor Operations Research
FedEx Freight utilizes cross docking operations in their hub-and-spoke terminals. Cross docking is a practice used to move freight directly from inbound trailers to outbound trailers with little to no storage in between. Currently, FedEx unloads inbound trailers using a work assignment method known as trailer-at-a-time, where one dockworker is assigned one trailer to unload all of its contents by forklift, and move them to their respective outbound trailer. This method results in about 40-45% of the forklift travel being categorized as “empty travel,” where no freight is being moved. To maximize dock performance, we will compare the trailer-at-a-time assignment level to two alternatives: shipment-at-a-time, and handling unit-at-a-time. A trailer can be broken down into two categories, shipments and handling units. A trailer can have multiple shipments, and each shipment can have multiple handling units. A handling unit is a single pallet or unit that needs to be moved. The shipment-at-a-time work assignment level assigns a worker and a forklift to an individual shipment within a trailer and moves all the handling units in the shipment to their outbound trailer. The worker then goes to the nearest inbound trailer and moves another shipment to its outbound trailer. The handling unit-at-a-time work assignment level assigns a worker and forklift to one unit, moves the unit to its outbound trailer and then goes to the nearest inbound trailer and moves to another handling unit.
Enhancing Preventive Maintenance Policy and Performing Asset Failure Analysis
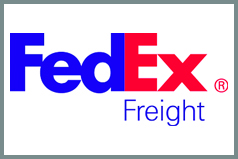
Team Members: David Wellendorf, Project Manager; Parker Fitzgerald, and Markham Roberson.
Industry Partner: FedEx Freight
Industry Contact: Ankit Sikka, Advisor-Operations Research
Many factors affect a company’s fleet availability in the transportation industry. Fleet availability refers to the operational proportion of a company’s total assets versus the proportion down for maintenance. Individually, factors necessitating asset maintenance may seem insignificant; however, their combined effects can have a significant negative impact on fleet availability. In order to increase availability, it is critical to understand asset failures and the driving reasons behind those failures. As a result, we have analyzed FedEx Freight’s equipment fleet to mathematically model asset failure and to improve the preventive maintenance policy, proactively supporting the identification of potential issues while maximizing fleet availability. Preventive maintenance is scheduled at regular intervals to service assets before a physical failure occurs. Corrective maintenance is any unexpected maintenance an asset requires due to an operational failure. Assets deteriorate as a function of usage and time. Preventive maintenance introduces a concept vital to the transportation industry: virtual age. This idea results in the extension of a machine’s useful life because it is running on newer components—and therefore a more efficient state, effectively reducing its age. We worked with maintenance data from the past five years to conduct risk analysis on the fleet to model its performance over time and to identify potential cost savings opportunities realizable through the enhancement of FedEx’s preventive maintenance policy. The team’s work will be used to make the company an even stronger competitor in the less-than-truckload shipping industry.
Optimizing Warehouse Toted Product Flow
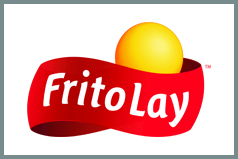
Team Members: Emerald Cole, Project Manager; Celia Aguilar, Daniel Gomez, and Clay Nivens.
Industry Partner: Frito-Lay
Industry Contacts: David Allen, Senior Site Director and Buddy Carroll, Logistics, Distribution and Transportation Director
We are working with a Frito-Lay production facility in Jonesboro, Arkansas. Our goal is to help increase the efficiency of the movement of their “toted” products, or products temporarily stored in large tote boxes, within the plant using either Automated Guided Vehicles (AGVs), manual forklifts or a combination. Frito-Lays toted products such as Cheetos® are mixed with other toted products such as Fritos® to make “mixed” products such as Munchies® and Cheetos® Mix Ups™. We are focused on moving the toted products within the facility from the production line or receiving dock to their designated storage area and finally to the mixing stations. The AGVs and the manual forklifts are both used to move finished products to the shipping dock in addition to moving the toted products around. The facility has one main corridor for all machinery to move products from the production side to the storage and shipping side. The AGVs in current use have been there for ten years but only recently in this capacity. As the Jonesboro facility has recently renovated and increased the size of their facility by twenty-five percent (25%), the newly designed parts of the building are not mapped out for AGV use. The primary issues we are facing include creating a better traffic flow within the main corridor as well as finding a way to work with the forklifts in the most efficient ways while minimizing costs.
Recommend an Ideal Fleet Mix of Asset and Non-asset Fleets to J.B. Hunt Management
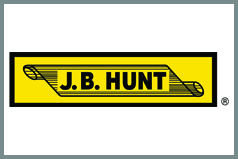
Team Members: Emily May, Project Manager; Lujain Rawwagah, Nick Taulbee, and Evan Tillman.
Industry Partner: J.B. Hunt Transport, Inc.
Industry Contact: Emily West, Logistics Engineer and Jeff Young, Engineering Manager
This IE Capstone Experience project was partnered with J.B. Hunt Transport Inc. Our goal was to recommend an ideal fleet mix of asset and non-asset fleets for J.B Hunt’s truckload business segment by minimizing cost and service failures. The significant differences between asset and non-asset fleets are an asset fleet consists of a J.B. Hunt driver, tractor, and trailer, while non-asset fleets only use a J.B. Hunt trailer. This results in a high capital investment for asset fleets because they are using J.B. Hunt equipment, and a lower capital investment for non-asset fleets. Additionally, asset fleets have forced dispatch, which means they cannot deny a load. Non-asset fleets have no forced dispatch, which means they can deny a load. In addition, there are two types of non-asset fleets, independent contract (IC) and power capacity solution (PCS). After analyzing historical data, we established that a significant component of recommending a fleet mix is incorporating denied loads when measuring service failures for each fleet type. In order to account for denied loads, we developed a tool adding denied loads into the service failure calculations. This tool will allow J.B. Hunt to change input parameters as more denied load data becomes available. In addition, we have provided an optimization tool that will allow J.B. Hunt to test several fleet mix percentage scenarios considering cost, service failures, and load demand. Lastly, we have provided recommendations to J.B. Hunt on how to continue this project in the future with more detailed and expanded data.
Reducing Tractor Cycle Times by Effectively Scheduling Maintenance Technicians
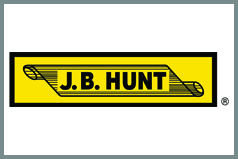
Team Members: Daniel Britton, Project Manager; John Tlapek, and Logan Weaver
Industry Partner: J.B. Hunt Transport, Inc.
Industry Contacts: Larry Lloyd, Engineering Manager, J.D. Ford, Logistics Engineer and Chuck Cameron, Director of Engineering
This project involves creating tractor technician schedules for J.B. Hunt Maintenance shops that will reduce overall tractor cycle times. At many of the shops, the day of the week with the most technicians scheduled and the day with the most tractors arriving at the shop are not the same; this misalignment is causing tractors to sit idly until a technician becomes available. Through the use of a Microsoft Excel tool, J.B. Hunt maintenance engineers will now be able to quickly create a technician schedule for any shop they desire. An Arena simulation model will then use this new technician schedule and data from the specified shop to simulate how tractor cycle times will be affected. These tools will allow J.B. Hunt Maintenance engineers to examine how technician schedules will affect tractor cycles before implementing them.
Statistical Process Control for Anomalies in Driver Pay
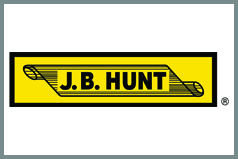
Team Members: Alex Schussler, Project Manager; Lexi Gaddy, and Chris Oliver
Industry Partner: J.B. Hunt Transport, Inc.
Industry Contacts: Alex Wong, Associate Logistics Engineer and Deric Bidwell, Engineering Manager
Financial auditing is an important process that can be used to identify anomalies in payroll. Drivers at J.B. Hunt are paid using an activity-based pay system. This means pay is determined by several factors: miles driven, hours on duty, and time spent on miscellaneous activities known as exceptions. Examples of exception pay are when a truck breaks down, a truck has to be cleaned for a load and having to wait an excessive amount of time at a customer. In these cases, the driver is compensated for the time he spends during each of these activities. Due to the lack of standardization in exception pay, auditing is tedious for the managers. The objective for this project is to design a decision support tool for auditing exception pay. Created using the coding language Python, this tool reads payroll data and generates sets of upper and lower limits. These sets are broken down by type of exception pay and the group to which the driver belongs. Using these limits, the tool then reads in the payroll data of the drivers and checks to make sure their pay is within the respective limits. If it is not, the tool exports a spreadsheet containing the potential outliers within the payroll data. This pinpoints where the managers should look while auditing. Along with flagging potential outliers, analysis is performed to identify whether the group a driver is in affects the amount of exception pay received.
Operator Scheduling Tool for Volatile Processes
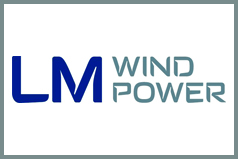
Team Members: Shadow Holcomb, Project Manager; Ardraya McCoy, Darius Jordan, and Zachary Willis.
Industry Partner: LM Wind Power
Industry Contact: Erin Lanford: Industrial Projects Manager
LM Wind Power faced the challenge of adequately scheduling workers for their post molding process. The post molding process is broken into three subsections that include: cut and grind, finishing, and assembly. This process makes up the bottleneck of the overall blade making process causing the push for more control. In response, time was dedicated to determining the root cause of the scheduling issues and analyzing past data of cycle times and performance measures used by the company. As a result, a tool was created that allowed LM Wind Power to provide a set of inputs that would be used to generate the number of workers and shift patterns required to produce a given number of turbine blades over a specified time period. Along with the design of this tool, suggestions were made to LM Wind Power for detailed data tracking metrics that should be implemented in order to get the most valuable and accurate usage of the tool going forward.
Reducing the Cycle Time of the Infusion Preparation Process
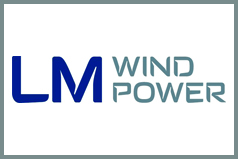
Team Members: Jacob Brosh, Project Manager; Cynthia Bocanegra, Ross Fulmer, and Cam Tu Nguyen
Industry Partner: LM Wind Power
Industry Contacts: Erin Lanford, Manager, Cycle Time Projects and Anthony Nguyen, Manager, Utilization Projects
For this project, our team has partnered with LM Wind Power, one of the world’s largest manufacturers of wind turbine blades. The making of a wind blade requires many manufacturing processes. This project specifically focuses on one, the infusion preparation process. The goals of this project have been to reduce the cycle time of this process, while maintaining or improving quality, and to develop a tool to aid in later process improvement. As part of a new big data initiative at LM, the company has been rolling out a new data collection tool formally known as “Cycle Time Tracker”. With this tool comes increased granularity in their data which our team has been able to leverage to expand upon a previous tool used at LM. With our new tool, production managers will be able to track learning rates at the subprocess level and determine if they are meeting the target production rates. One of the challenges we have faced is adapting to the range of blade sizes produced by LM. Understanding the relationship between blade size and cycle times was crucial in extrapolating any process changes as well as developing our decision support tool. While our team has been working specifically with LM’s Little Rock facility, we expect to see the changes that result from this project implemented across their thirteen facilities worldwide.
Laying the Foundation for Standardized Work at Tyson Mexican Original
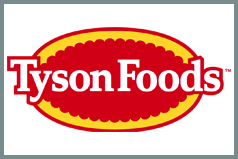
Team Members: Sarah Kiner, Project Manager; Adam Hope, Liz Luzcando, and Arturo Nunez
Industry Partner: Tyson Foods, Inc.
Industry Contacts: Marc Schwartz, Sr. Continuous Improvement Manager, Dan Doughty, Sr. Business Engineer and Travis Hartley, Sr. Business Engineer
The main focus of this project was to calculate a 6 x 6 matrix solution and the development of supportive standard work instructions. At Tyson’s Mexican Original Plant located in Fayetteville Arkansas, a new product has been developed for the facility’s largest customer (Taco Bell) and will be produced at very high volume (nearly 400,000 pieces per day). Preliminary engineering tests on production equipment were successful at a 68% quality rate. However, scrap volume was 32%, which was unacceptable given the current scrap benchmark on similar equipment was ~ 1% with an overall plant average of 2-4%. Our team was tasked to develop a mathematical model and associated standard operating procedures based on data developed from actual production equipment currently on-line. Using a standardized measurement process, we created a forecasting model with supporting standard operating procedures to develop a matrix of desired size tortillas from specific dough ball weights. The final analysis also supports operator training and repeatability by following a standardized process. From the baseline volume, each 10%-point reduction in scrap from 32% to 2% equates to an annualized cost reduction of about $630K. Attaining the full 30% improvement is a $1.9MM cost avoidance. Working as a team and with Tyson’s production leaders, our group was able to apply the interdisciplinary techniques learned in the classroom to real life problems. A series of lessons learned will be discussed in the paper, primarily focusing on the importance of establishing a common understanding across business units and across production shifts.
Improving Process Monitoring for Tyson Foods, Berry Street
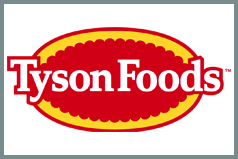
Team Members: Grant Holman, Project Manager; Justo Barrios, and Hans Maggio.
Industry Partner: Tyson Foods, Inc.
Industry Contacts: Jeremy Hank, Continuous Improvement Engineer and Thomas Bond, Senior Manager Continuous Improvement
The Berry Street facility, located in Springdale Arkansas, is one of Tyson Foods Inc.’s premiere poultry production facilities. Focusing on Line 1: Further Processing within the plant, this project targets the current process monitoring system. The current system is paper based, resulting in both incomplete and missing records. In order to standardize and improve this process, our group has computerized line 1’s process monitoring. Using a coding platform called Django, we created a web-based tool to collect and archive data. This tool mirrors the paper process but allows the data to be stored in an organized fashion for later analysis. Increasing yield on Line 1 is the main objective of this project, and based on research, computerizing the process monitoring system should result in increases and therefore cost savings for Tyson. Since the beginning of our project, Tyson has begun to develop an enterprise-wide computerized process monitoring system called the Tyson Production System. This new system will incorporate process control as well and will generate cost savings across Tyson’s 20+ production facilities. As a secondary objective, we completed a simulation of Line 1 in Arena. This model was completed in order to quickly test any changes to the major processes in line with statistical backing. Through the completion of these two objectives, we believe we have given tremendous value to Tyson, which will later generate a large amount of cost savings.